Robot Plume Tracking Method Based on Reinforcement Learning in Continuous State Behavior Domain
A continuous state and reinforcement learning technology, applied in neural learning methods, instruments, manipulators, etc., can solve problems such as high cost, inability to adapt to the environment, and insufficient consideration
- Summary
- Abstract
- Description
- Claims
- Application Information
AI Technical Summary
Problems solved by technology
Method used
Image
Examples
Embodiment Construction
[0082] The robot plume tracking method based on reinforcement learning in the continuous state behavior domain proposed by the present invention will be further described in detail below in conjunction with the accompanying drawings and specific embodiments.
[0083] The robot plume tracking method based on continuous state behavior domain reinforcement learning proposed by the present invention describes the robot plume tracking process by using sequence decision-making. At the initial moment, the underwater robot will obtain the current deep-sea environmental information, including signals detectable by sensors such as the current velocity in the deep sea and the concentration of hydrothermal plume signals. Combine these signals into the state vector required for single-step path planning, input the state vector into the current decision-making neural network, and the decision-making neural network outputs the direction of the robot at this moment. After the robot runs at a c...
PUM
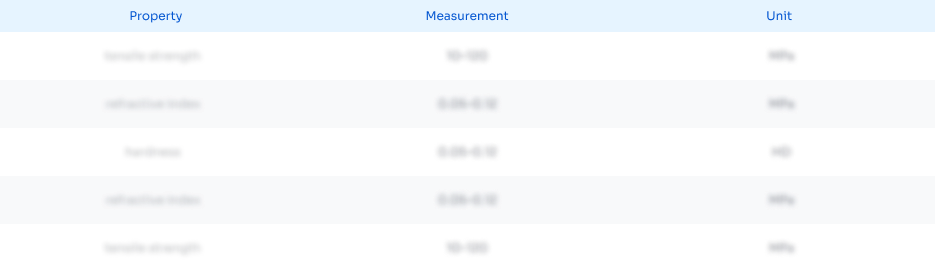
Abstract
Description
Claims
Application Information
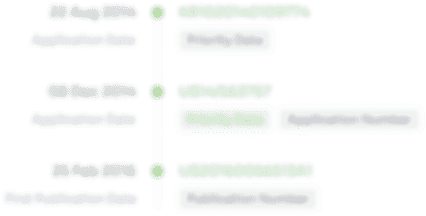
- R&D Engineer
- R&D Manager
- IP Professional
- Industry Leading Data Capabilities
- Powerful AI technology
- Patent DNA Extraction
Browse by: Latest US Patents, China's latest patents, Technical Efficacy Thesaurus, Application Domain, Technology Topic, Popular Technical Reports.
© 2024 PatSnap. All rights reserved.Legal|Privacy policy|Modern Slavery Act Transparency Statement|Sitemap|About US| Contact US: help@patsnap.com