Kernel extreme learning machine flood forecast method based on sparse self-encoding
A kernel extreme learning machine and sparse self-encoding technology, applied in neural learning methods, prediction, biological neural network models, etc., can solve the problems of complexity and less application of deep learning, and achieve the effect of improving the prediction effect.
- Summary
- Abstract
- Description
- Claims
- Application Information
AI Technical Summary
Problems solved by technology
Method used
Image
Examples
Embodiment Construction
[0057] Such as figure 1 As shown, a flood forecasting method based on sparse self-encoding kernel extreme learning machine, including the following steps:
[0058] (1) Select the flood data of small and medium rivers, and organize and clean the data;
[0059] (2) Select appropriate predictors and arrange samples, and preprocess the sample data;
[0060] (3) The original sample data is subjected to unsupervised learning through a multi-layer sparse autoencoder, and the optimal network layer parameters are trained respectively;
[0061] (4) The sample data after multi-layer sparse self-encoding learning is used as the input of the KELM model, and the SAE_KELM model is constructed to predict and evaluate the corresponding results.
[0062] In order to improve the accuracy of flood forecasting for small and medium-sized rivers, this paper combines the sparse self-encoding technology and the kernel extreme learning machine model to construct a deep network flood forecasting model...
PUM
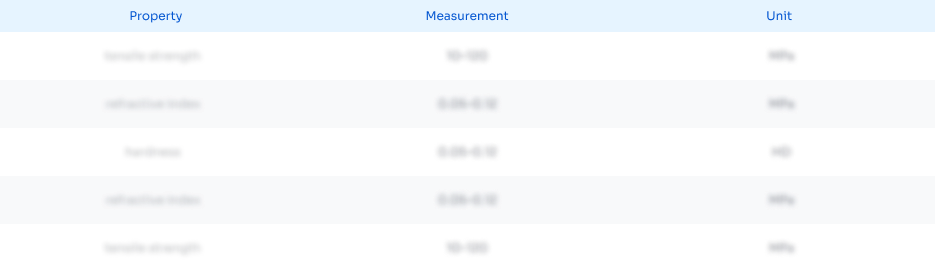
Abstract
Description
Claims
Application Information
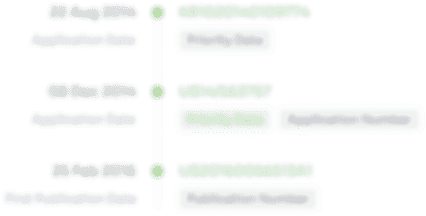
- R&D Engineer
- R&D Manager
- IP Professional
- Industry Leading Data Capabilities
- Powerful AI technology
- Patent DNA Extraction
Browse by: Latest US Patents, China's latest patents, Technical Efficacy Thesaurus, Application Domain, Technology Topic, Popular Technical Reports.
© 2024 PatSnap. All rights reserved.Legal|Privacy policy|Modern Slavery Act Transparency Statement|Sitemap|About US| Contact US: help@patsnap.com