Classification and identification method of power quality multi-disturbance signals based on ga-svm
A disturbance signal, classification and identification technology, applied in the direction of measuring electrical variables, measuring electricity, measuring devices, etc., can solve the problems of easy modal aliasing, easy failure of extracted feature quantities, and long training time.
- Summary
- Abstract
- Description
- Claims
- Application Information
AI Technical Summary
Problems solved by technology
Method used
Image
Examples
Embodiment Construction
[0047] Below in conjunction with accompanying drawing and embodiment the specific scheme of the present invention is further described, asfigure 1 As shown, a GA-SVM-based classification and identification method for power quality multi-disturbance signals, specifically includes the following steps:
[0048] Step A: Use the voltage sensor to collect the voltage signal in the power grid, and use the improved EEMD-HHT method to perform feature extraction on the collected voltage signal, specifically including steps A1-A4.
[0049] Step A1: Use the voltage sensor to collect the voltage signal in the power grid, and then use the signal conditioning circuit to filter and shape the collected signal; use the voltage sensor to collect the voltage signal in the power grid and use the signal conditioning circuit to filter the collected signal Both the processing and the shaping process belong to the prior art, and will not be described too much here.
[0050] Step A2: Transfer the filte...
PUM
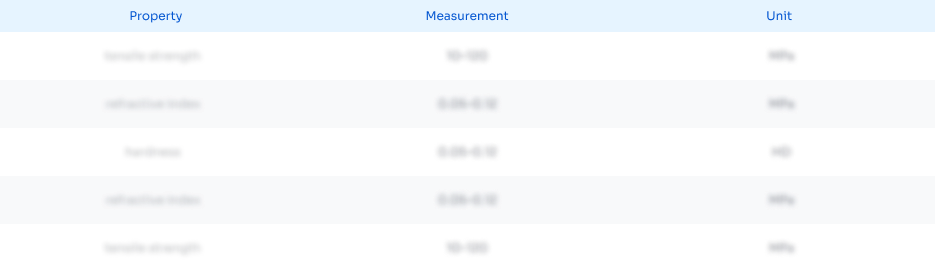
Abstract
Description
Claims
Application Information
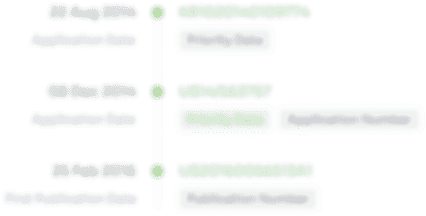
- R&D Engineer
- R&D Manager
- IP Professional
- Industry Leading Data Capabilities
- Powerful AI technology
- Patent DNA Extraction
Browse by: Latest US Patents, China's latest patents, Technical Efficacy Thesaurus, Application Domain, Technology Topic, Popular Technical Reports.
© 2024 PatSnap. All rights reserved.Legal|Privacy policy|Modern Slavery Act Transparency Statement|Sitemap|About US| Contact US: help@patsnap.com