Polarized SAR classification method based on deep learning of shallow-layer characteristics and T-matrix
A technology of deep learning and classification methods, applied in the field of image processing, can solve problems such as the impact of classification results, insufficient image expression, and increased workload of scientific researchers, and achieve good classification, accurate classification results, and rich information
- Summary
- Abstract
- Description
- Claims
- Application Information
AI Technical Summary
Problems solved by technology
Method used
Image
Examples
Embodiment Construction
[0024] The embodiments and effects of the present invention will be further described in detail below in conjunction with the accompanying drawings.
[0025] refer to figure 1 , the implementation steps of the present invention are as follows:
[0026] Step 1. Perform filtering processing on the original polarimetric SAR image.
[0027] Input the polarimetric SAR image to be classified, and use the refined polarimetric Lee filter in polSARpro_v4.0 software to remove the speckle noise in the image to be classified through a sliding window of 7×7 pixels, and obtain the filtered polarimetric SAR image.
[0028] Step 2. Extract the polarimetric shallow features of the filtered polarimetric SAR image.
[0029] The existing common methods for extracting polarization shallow features include Freeman decomposition and Cloude decomposition. In this example, the Cloude decomposition method is used to extract polarization shallow features from the filtered polarimetric SAR image. The ...
PUM
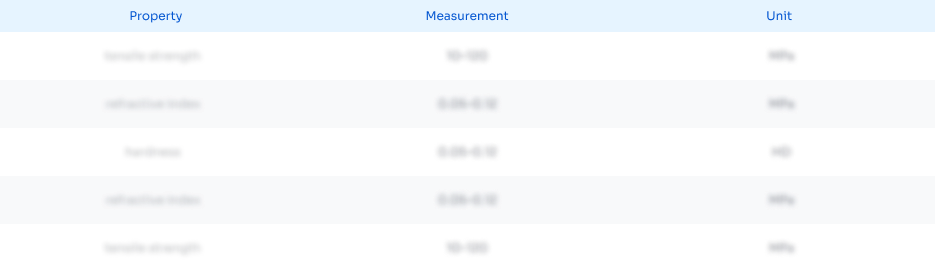
Abstract
Description
Claims
Application Information
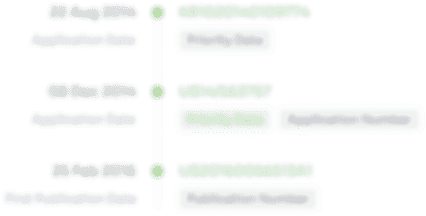
- R&D Engineer
- R&D Manager
- IP Professional
- Industry Leading Data Capabilities
- Powerful AI technology
- Patent DNA Extraction
Browse by: Latest US Patents, China's latest patents, Technical Efficacy Thesaurus, Application Domain, Technology Topic, Popular Technical Reports.
© 2024 PatSnap. All rights reserved.Legal|Privacy policy|Modern Slavery Act Transparency Statement|Sitemap|About US| Contact US: help@patsnap.com