Identification method of primary central nervous system lymphoma and glioblastoma based on sparse representation system
A glioblastoma, sparse representation technology, used in character and pattern recognition, computer parts, image data processing, etc.
- Summary
- Abstract
- Description
- Claims
- Application Information
AI Technical Summary
Problems solved by technology
Method used
Image
Examples
Embodiment Construction
[0044] The following are the specific implementation steps of the whole method:
[0045] 1. Firstly, perform brain removal and gray-scale normalization operations on the images in the data set, and select 40 images from the T1-enhanced images and T2-weighted image sets for manual labeling of tumor areas, and then send the labeling results and corresponding images to Two kinds of convolutional neural networks constructed were used to train the network parameters, and finally the two trained convolutional neural networks were used to segment the tumor area of the corresponding modality image.
[0046] 2. Extract the set of image blocks contained in the tumor area, the size of the image blocks is 11*11, and the center interval of the image blocks is 5*5. For T1-enhanced modal images, select the image block sets corresponding to 20 cases of primary brain lymphoma images, use the K singular value decomposition method to train the primary brain lymphoma dictionary, and select 20 c...
PUM
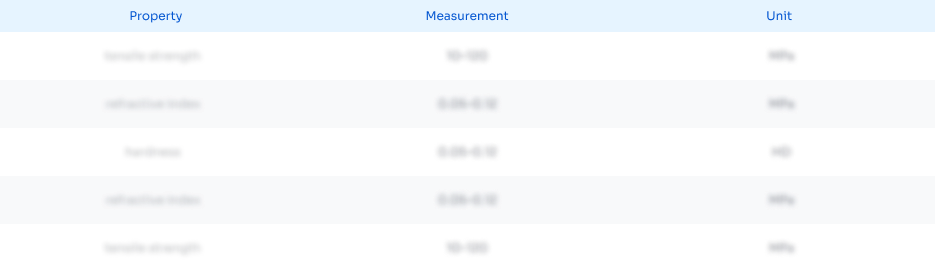
Abstract
Description
Claims
Application Information
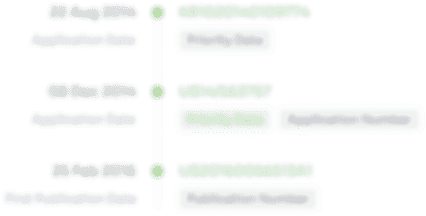
- R&D Engineer
- R&D Manager
- IP Professional
- Industry Leading Data Capabilities
- Powerful AI technology
- Patent DNA Extraction
Browse by: Latest US Patents, China's latest patents, Technical Efficacy Thesaurus, Application Domain, Technology Topic, Popular Technical Reports.
© 2024 PatSnap. All rights reserved.Legal|Privacy policy|Modern Slavery Act Transparency Statement|Sitemap|About US| Contact US: help@patsnap.com