Flexible job shop batch scheduling method based on genetic algorithm
A technology of flexible operation and genetic algorithm, applied in the direction of genetic rules, calculation, genetic model, etc., can solve the problems of increased complexity, high complexity, and complex problems of evolutionary operations
- Summary
- Abstract
- Description
- Claims
- Application Information
AI Technical Summary
Problems solved by technology
Method used
Image
Examples
Embodiment 1
[0082] Embodiment one: as attached figure 1 As shown, the operation steps of this flexible workshop batch scheduling method based on genetic algorithm are as follows:
[0083] Step 1, determine the operating parameters.
[0084] Step 2, initial population generation.
[0085] Step 3, individual fitness calculation.
[0086] Step 4, select an operation.
[0087] Step five, cross operation.
[0088] Step six, mutation operation.
[0089] Step seven, terminate the judgment.
Embodiment 2
[0090] Embodiment two: see Figure 1 to Figure 5 , the present embodiment is basically the same as Embodiment 1, and the special features are as follows:
[0091] The operating parameters are population size M, crossover probability P C , the mutation probability P M and the number of iterations T.
[0092] When the initial population is generated, a segmented coding method is used to obtain batch codes and process codes to generate individuals, and the batch codes are generated using a roulette method.
[0093] When calculating the individual fitness, select machines for each batch of workpieces according to the earliest completion criterion of the workpiece, calculate the time, obtain the total completion time, and then take the reciprocal of the total completion time as the fitness value; obviously, the shorter the completion time, the higher the fitness value. high.
[0094] The selection operation adopts a roulette selection method, and the selection probability depen...
Embodiment 3
[0098] Embodiment 3: This embodiment is basically the same as Embodiment 1, and the special features are as follows:
[0099] The specific details of each step are as follows:
[0100] Step 1. Determine the operating parameters
[0101] The operating parameters of the genetic algorithm include population size M, crossover probability P C , the mutation probability P M , the number of iterations T. The population size M is generally 20-100, and the crossover probability P C Generally take 0.4 ~ 0.99, the variation probability P M Generally, it is 0.0001~0.1, and the number of iterations T is generally 100~500.
[0102] Step 2. Initial Population Generation
[0103] The generation of the initial population depends on coding. The coding of the flexible job shop batch scheduling problem needs to contain two parts of information. The first part is the batch information of each workpiece, which determines how many batches each workpiece is divided into. The number of workpiece...
PUM
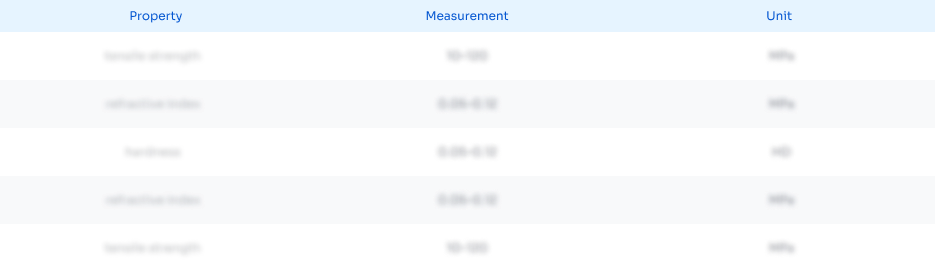
Abstract
Description
Claims
Application Information
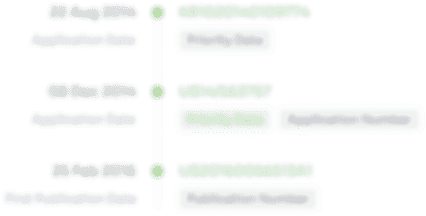
- R&D Engineer
- R&D Manager
- IP Professional
- Industry Leading Data Capabilities
- Powerful AI technology
- Patent DNA Extraction
Browse by: Latest US Patents, China's latest patents, Technical Efficacy Thesaurus, Application Domain, Technology Topic, Popular Technical Reports.
© 2024 PatSnap. All rights reserved.Legal|Privacy policy|Modern Slavery Act Transparency Statement|Sitemap|About US| Contact US: help@patsnap.com