Large-scale face recognition method based on depth convolution neural network model
A neural network model and deep convolution technology, applied in the fields of artificial intelligence and computer vision, can solve problems such as large-scale face recognition difficulties, and achieve the effect of solving complex model construction, excellent results, and simple model structure.
- Summary
- Abstract
- Description
- Claims
- Application Information
AI Technical Summary
Problems solved by technology
Method used
Image
Examples
Embodiment Construction
[0050] The specific implementation of the present invention, the deep convolutional neural network model based on residual learning in the million-level face recognition task is explained below in conjunction with the accompanying drawings, and the specific operation steps of the realization are as attached figure 1 shown.
[0051] 1. Preprocess the image
[0052] First use the image processing tool MTCNN [19] Detect the face in the picture, then use MTCNN to detect 5 key points in the face (two eyes, nose tip, corners of mouth on both sides), and then face alignment method [20] Face alignment is performed, and finally the size of the processed image is normalized to 112×96.
[0053] 2. Build the deep convolutional neural network model based on residual learning proposed by the present invention
[0054] Using the deep learning framework Caffe, build the deep convolutional neural network model based on residual learning proposed by the present invention, as shown in the att...
PUM
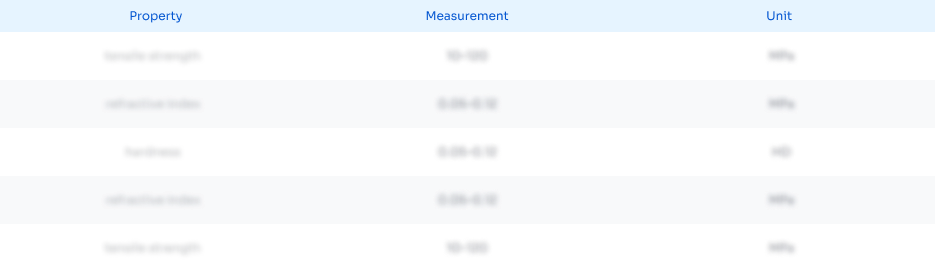
Abstract
Description
Claims
Application Information
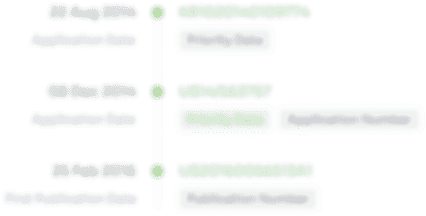
- R&D Engineer
- R&D Manager
- IP Professional
- Industry Leading Data Capabilities
- Powerful AI technology
- Patent DNA Extraction
Browse by: Latest US Patents, China's latest patents, Technical Efficacy Thesaurus, Application Domain, Technology Topic, Popular Technical Reports.
© 2024 PatSnap. All rights reserved.Legal|Privacy policy|Modern Slavery Act Transparency Statement|Sitemap|About US| Contact US: help@patsnap.com