Neural network-based acoustic glass defect detection method
A neural network and glass defect technology, which is applied in the field of acoustic glass defect detection based on neural network, can solve problems such as difficulty in unifying judgment standards, poor correlation, and unsatisfactory results
- Summary
- Abstract
- Description
- Claims
- Application Information
AI Technical Summary
Problems solved by technology
Method used
Image
Examples
Embodiment Construction
[0030] A neural network-based acoustic glass defect detection method of the present invention will be described in detail below in combination with embodiments and drawings.
[0031] Such as figure 1 Shown, a kind of acoustic glass defect detection method based on neural network of the present invention comprises the following steps:
[0032] 1) Use the pickup to collect the knocking signal of the glass sample in the actual production environment;
[0033] 2) Due to the noise and other unavoidable factors in the environment, there are noise and abnormal signals in the collected data. In order to obtain a pure tapping signal, it is necessary to preprocess the collected tapping signal, including noise reduction, endpoint detection and removal of abnormal data, to obtain a pure tapping signal. , wherein, a high-pass filter is used for noise reduction, and a double-threshold algorithm of short-term energy and short-term average zero-crossing rate is used to detect the endpoint o...
PUM
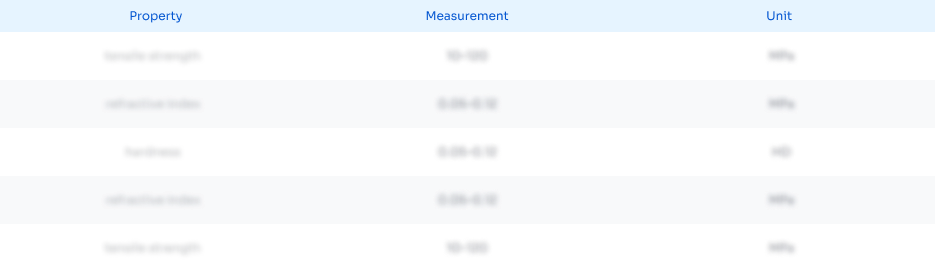
Abstract
Description
Claims
Application Information
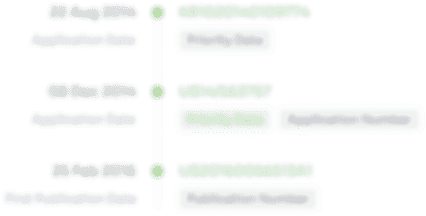
- R&D Engineer
- R&D Manager
- IP Professional
- Industry Leading Data Capabilities
- Powerful AI technology
- Patent DNA Extraction
Browse by: Latest US Patents, China's latest patents, Technical Efficacy Thesaurus, Application Domain, Technology Topic, Popular Technical Reports.
© 2024 PatSnap. All rights reserved.Legal|Privacy policy|Modern Slavery Act Transparency Statement|Sitemap|About US| Contact US: help@patsnap.com