Prediction model based on optimized extreme learning machine (ELM)
An extreme learning machine and predictive model technology, applied in neural learning methods, biological neural network models, neural architectures, etc., can solve problems such as application difficulties, high requirements for independent variable data, and inability to solve multi-category factor fitting, etc. Solving the effect of few predictors and relaxing data linearization constraints
Inactive Publication Date: 2017-05-10
ZHENGZHOU UNIV
View PDF0 Cites 19 Cited by
- Summary
- Abstract
- Description
- Claims
- Application Information
AI Technical Summary
Problems solved by technology
[0003] Under the current conditions of complicated pathogenic factors, the above regression analysis strategy cannot solve the problem of multi-classification factor fitting.
In addition, probabilistic strategies have high requirements for independent variable data, and it is difficult to apply them in the absence of large-scale cross-sectional research data
Method used
the structure of the environmentally friendly knitted fabric provided by the present invention; figure 2 Flow chart of the yarn wrapping machine for environmentally friendly knitted fabrics and storage devices; image 3 Is the parameter map of the yarn covering machine
View moreImage
Smart Image Click on the blue labels to locate them in the text.
Smart ImageViewing Examples
Examples
Experimental program
Comparison scheme
Effect test
Embodiment
[0071] In order to illustrate the technical effects of the present invention, a specific application example is used to verify the implementation of the present invention.
the structure of the environmentally friendly knitted fabric provided by the present invention; figure 2 Flow chart of the yarn wrapping machine for environmentally friendly knitted fabrics and storage devices; image 3 Is the parameter map of the yarn covering machine
Login to View More PUM
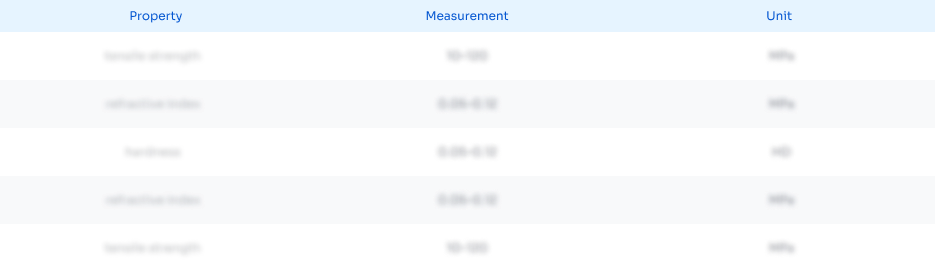
Abstract
The invention discloses a cardiovascular disease (CVD) prediction model using an extreme learning machine (ELM) algorithm based on single-hidden layer feed-forward neural networks (SLFNs), which means an ELPSO-ELM hybrid intelligent algorithm prediction model. The ELPSO-ELM hybrid intelligent algorithm prediction model is designed by using the algorithm through the following steps: building a leadership particle enhanced particle swarm optimization algorithm (ELPSO) by carrying out five stages of continuous variation modes, which are respectively the Gaussian variation, Cauchy variation, Pg each dimension opposition-based variation, Pg overall opposition-based variation and DE-based variation; and optimizing the hidden layer unit parameters of the SLFNs by using the optimization strategy of the particle swarm optimization (PSO) algorithm. According to the ELPSO-ELM hybrid intelligent algorithm prediction model disclosed by the invention, the limitations of traditional models which contain less prediction factors and have high data requirements can be solved.
Description
technical field [0001] The invention relates to the field of prediction models, in particular to a prediction model based on an optimized extreme learning machine. Background technique [0002] At present, classic Framingham, SCORE, WHO and other models have achieved good prediction results in 10-year incidence risk prediction. The main modeling ideas include: the inference prediction method based on the trending reasoning based on the epidemic process and characteristics of the disease, and the qualitative prediction based on the knowledge level and subjective experience of experts on cardiovascular disease (CVD); large follow-up cohorts based on disease risk factors and incidence Mathematical forecasting methods for cross-sectional data, such as time series forecasting models and regression forecasting models, establish mathematical probability models for regularly changing data and quantitatively mine the proportional relationship between pathogenic factors, which require...
Claims
the structure of the environmentally friendly knitted fabric provided by the present invention; figure 2 Flow chart of the yarn wrapping machine for environmentally friendly knitted fabrics and storage devices; image 3 Is the parameter map of the yarn covering machine
Login to View More Application Information
Patent Timeline
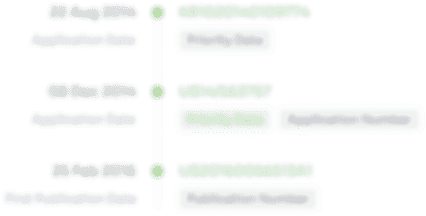
Patent Type & Authority Applications(China)
IPC IPC(8): G06N3/04G06N3/08
CPCG06N3/04G06N3/08
Inventor 逯鹏郭赛迪林予松李奇航刘豪杰牛新张景景张行进
Owner ZHENGZHOU UNIV
Who we serve
- R&D Engineer
- R&D Manager
- IP Professional
Why Patsnap Eureka
- Industry Leading Data Capabilities
- Powerful AI technology
- Patent DNA Extraction
Social media
Patsnap Eureka Blog
Learn More Browse by: Latest US Patents, China's latest patents, Technical Efficacy Thesaurus, Application Domain, Technology Topic, Popular Technical Reports.
© 2024 PatSnap. All rights reserved.Legal|Privacy policy|Modern Slavery Act Transparency Statement|Sitemap|About US| Contact US: help@patsnap.com