Image classification method based on deep ridgelet neural network
A neural network and classification method technology, applied in the field of intelligent image processing, can solve the problems of reducing data, difficult to satisfy image data, and difficult to achieve the optimal solution, etc., to achieve the effect of increasing the approximation rate, improving the classification accuracy, and improving the sparse approximation ability
- Summary
- Abstract
- Description
- Claims
- Application Information
AI Technical Summary
Problems solved by technology
Method used
Image
Examples
Embodiment Construction
[0039] The technical solutions and effects of the present invention will be described in further detail below with reference to the accompanying drawings.
[0040] refer to figure 1 , the implementation steps of the present invention are as follows:
[0041] Step 1, obtain training samples and test samples.
[0042] Randomly select 10% of all image samples from the image library as the training image sample set, wherein the nth training image sample is recorded as P(n), n=1,..., N is the number of training image samples, the first The class label of n training image samples is L(n), and the remaining samples are used as test image samples, Q(m) is the mth test image sample, m=1,...,M, M is the number of test image samples number.
[0043] Step 2, regroup the training samples.
[0044] For each training image sample P(n), rearrange it into a column vector S according to the row-first rule 1 (n):
[0045]
[0046] in, represents the column vector S 1 The i-th element...
PUM
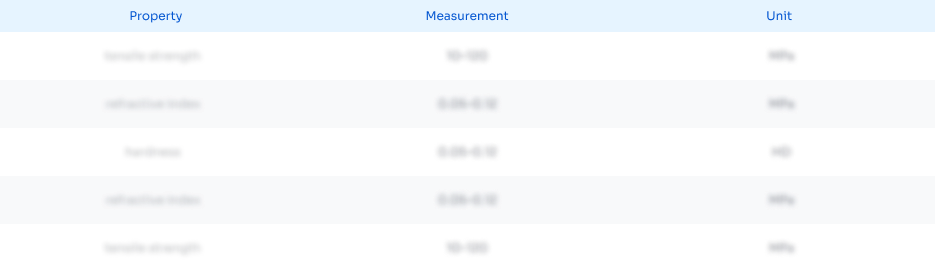
Abstract
Description
Claims
Application Information
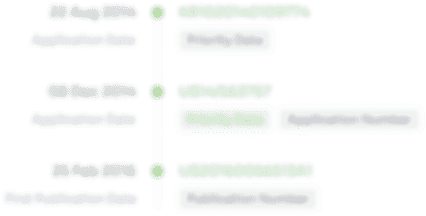
- R&D Engineer
- R&D Manager
- IP Professional
- Industry Leading Data Capabilities
- Powerful AI technology
- Patent DNA Extraction
Browse by: Latest US Patents, China's latest patents, Technical Efficacy Thesaurus, Application Domain, Technology Topic, Popular Technical Reports.
© 2024 PatSnap. All rights reserved.Legal|Privacy policy|Modern Slavery Act Transparency Statement|Sitemap|About US| Contact US: help@patsnap.com