Multispectral remote sensing image terrain classification method based on deep and semi-supervised transfer learning
A transfer learning and remote sensing image technology, applied in the field of remote sensing image processing, can solve the problems of inconsistent distribution of training and testing samples, insufficient training samples, etc., and achieve the effect of improving classification accuracy, good classification effect, and preventing deviation.
- Summary
- Abstract
- Description
- Claims
- Application Information
AI Technical Summary
Problems solved by technology
Method used
Image
Examples
Embodiment Construction
[0057] The present invention provides a multi-spectral remote sensing image object classification method based on deep semi-supervised transfer learning. When the training samples are insufficient, the source domain similar to the target domain and the unmarked samples of the target domain are used to assist the target domain data for classification. Specifically: input the multi-spectral remote sensing image of the training sample, take out the training data set D and kNN data K1 and K2 according to the ground truth; divide the training data set D into two parts, and train two different CNN models CNN1 and CNN2 respectively; Classified multispectral images, two classification result graphs F1 and F2 are obtained in two CNN models; two kNN neighborhood algorithm graphs are constructed according to training samples K1 and K2, kNN1 and kNN2; test data A1 and F2 are taken out with F1 and F2 A2, use the kNN proximity algorithm to classify the data; update the classification result ...
PUM
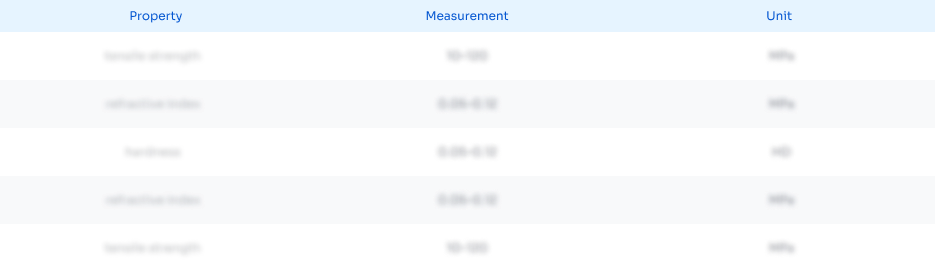
Abstract
Description
Claims
Application Information
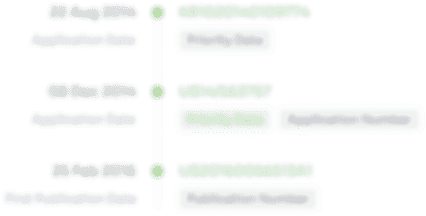
- Generate Ideas
- Intellectual Property
- Life Sciences
- Materials
- Tech Scout
- Unparalleled Data Quality
- Higher Quality Content
- 60% Fewer Hallucinations
Browse by: Latest US Patents, China's latest patents, Technical Efficacy Thesaurus, Application Domain, Technology Topic, Popular Technical Reports.
© 2025 PatSnap. All rights reserved.Legal|Privacy policy|Modern Slavery Act Transparency Statement|Sitemap|About US| Contact US: help@patsnap.com