Detection method of variable-scale infrared small targets based on fast automatic density clustering
A technology of density clustering and detection methods, which is applied in the direction of instruments, computing, character and pattern recognition, etc., can solve the problems of high false positive rate, density clustering algorithm cannot automatically determine the cluster center and cannot overcome two types of errors in clustering and segmentation , low detection rate and other issues, to speed up, overcome exponential complexity problems, solve over-segmentation and under-segmentation effects
- Summary
- Abstract
- Description
- Claims
- Application Information
AI Technical Summary
Problems solved by technology
Method used
Image
Examples
Embodiment Construction
[0042] The implementation steps of the present invention mainly include: extraction of interest points based on SURF, fast automatic density clustering and backtracking algorithm.
[0043] Step 1: Synthesize the simulation image sequence F(t), (t=1,...N), refer to the synthesis method provided by the document "Infrared patch-imagemodel for small target detection in a single image.IEEE Trans.On Imageprocessing,2013" , where the background is the real cloud-sky or sea-sky background, the size of the embedded simulated small target is controlled between 3×3 pixels and 10×10 pixels, and the noise variance is controlled within the range of (0.01, 0.03).
[0044] Step 2: For the simulated image sequence F(t), (t=1,...N), the interest point extraction based on SURF is performed frame by frame.
[0045] (1) Save the grayscale image sequence F(t) to be detected in the folder F;
[0046] (2) set up a csv file called 'image coordinates' in folder F in step (1) with the fopen function of...
PUM
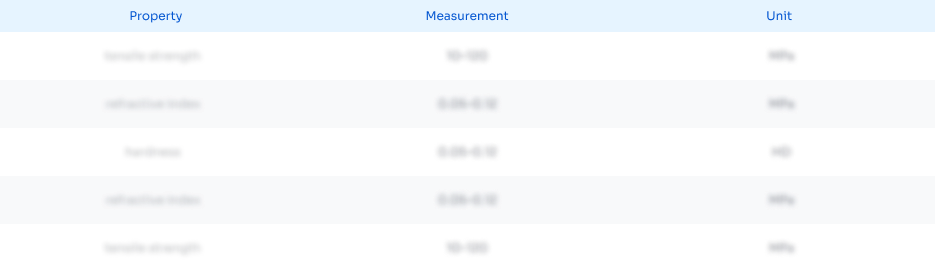
Abstract
Description
Claims
Application Information
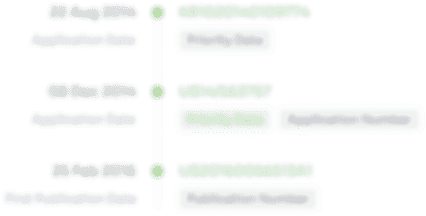
- R&D Engineer
- R&D Manager
- IP Professional
- Industry Leading Data Capabilities
- Powerful AI technology
- Patent DNA Extraction
Browse by: Latest US Patents, China's latest patents, Technical Efficacy Thesaurus, Application Domain, Technology Topic, Popular Technical Reports.
© 2024 PatSnap. All rights reserved.Legal|Privacy policy|Modern Slavery Act Transparency Statement|Sitemap|About US| Contact US: help@patsnap.com