An Improved Seat Belt Detection Method
A detection method and technology of seat belts, applied in neural learning methods, instruments, biological neural network models, etc., can solve the problems of single image noise, easy to be affected by external factors, and long training time.
- Summary
- Abstract
- Description
- Claims
- Application Information
AI Technical Summary
Problems solved by technology
Method used
Image
Examples
Embodiment 1
[0192] Embodiment 1 (under different methods, to the comparison of the recognition rate of image)
[0193]The test and training seatbelt image library in this example is the actual bayonet image, and the size of the image is 120*110 pixels. The experimental running platform is Lenovo 64-bit notebook, Intel i5 processor, CPU frequency 2.60GHz, 4G running memory. Multiple comparison algorithms are tested on the same hardware platform environment. The total sample library is 10,000, the number of samples used in the training library is 6,000, and the test library is 2,000.
[0194] Use three methods to detect seat belts, including: (1) Canny+adaboost training method (2) deep learning seat belt detection method (3) the method of the present invention, the recognition rate is shown in the following table:
[0195] Detection method
[0196] It can be seen from the table that the recognition accuracy of the method of the present invention is higher, followed by the deep l...
PUM
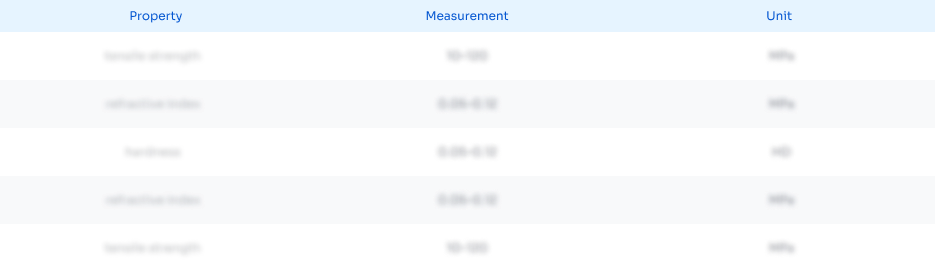
Abstract
Description
Claims
Application Information
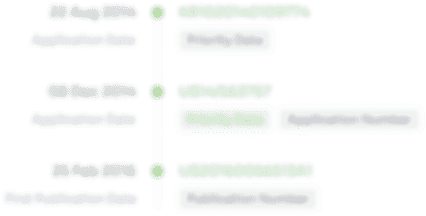
- Generate Ideas
- Intellectual Property
- Life Sciences
- Materials
- Tech Scout
- Unparalleled Data Quality
- Higher Quality Content
- 60% Fewer Hallucinations
Browse by: Latest US Patents, China's latest patents, Technical Efficacy Thesaurus, Application Domain, Technology Topic, Popular Technical Reports.
© 2025 PatSnap. All rights reserved.Legal|Privacy policy|Modern Slavery Act Transparency Statement|Sitemap|About US| Contact US: help@patsnap.com