Structure sparse multi-kernel learning-based multi-modal data feature screening and classification method
A data feature and multi-core learning technology, which is applied in character and pattern recognition, instruments, computer components, etc., to achieve the effect of simple and compact model and reduce model complexity
- Summary
- Abstract
- Description
- Claims
- Application Information
AI Technical Summary
Problems solved by technology
Method used
Image
Examples
Embodiment Construction
[0041]The present invention will be further described below in conjunction with the accompanying drawings and specific embodiments. It should be understood that the preferred embodiments described here are only used to illustrate and explain the present invention, and are not intended to limit the present invention.
[0042] As a specific example, the data of senile dementia are adopted as follows. The data include nuclear magnetic resonance data (Nuclear Magnetic Resonance Imaging, MRI for short) and positron emission computed tomography data (Positron emission tomography, PET for short). The purpose is to use MRI Two types of medical imaging data, PET and PET, are used to diagnose whether an individual suffers from Alzheimer's disease, such as figure 1 What is shown describes the problems to be solved in this embodiment. The data contains image data of 90 individuals, and each individual data contains data of both MRI and PET modalities; in this data, there are 42 individual...
PUM
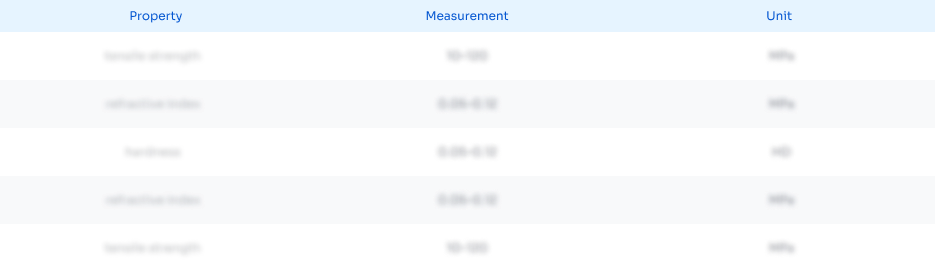
Abstract
Description
Claims
Application Information
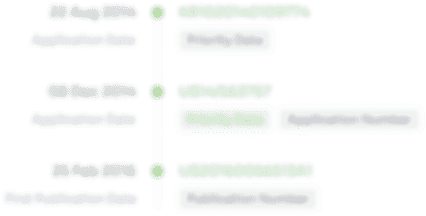
- Generate Ideas
- Intellectual Property
- Life Sciences
- Materials
- Tech Scout
- Unparalleled Data Quality
- Higher Quality Content
- 60% Fewer Hallucinations
Browse by: Latest US Patents, China's latest patents, Technical Efficacy Thesaurus, Application Domain, Technology Topic, Popular Technical Reports.
© 2025 PatSnap. All rights reserved.Legal|Privacy policy|Modern Slavery Act Transparency Statement|Sitemap|About US| Contact US: help@patsnap.com