Wavelet transform-based fine-granularity self-learning integration prediction method
A technology of wavelet transform and prediction method, applied in informatics, special data processing applications, instruments, etc., can solve the problems of low prediction accuracy, weak generalization ability, and no consideration of time characteristics, etc., to achieve good robustness and accuracy The effect of predicting performance
- Summary
- Abstract
- Description
- Claims
- Application Information
AI Technical Summary
Problems solved by technology
Method used
Image
Examples
no. 1 Embodiment
[0067] For the first specific embodiment, the method is used for air quality prediction, and the output features and input features include pollution features, meteorological features, and the like.
[0068] S32. Extracting highly relevant features.
[0069] The degree of linear relationship between variables is usually described by the correlation coefficient r, for example:
[0070] |r|>0.95 there is a significant correlation;
[0071] |r|≥0.8 highly correlated;
[0072] 0.5≤|r|<0.8 Moderate correlation;
[0073] 0.3≤|r|<0.5 low correlation;
PUM
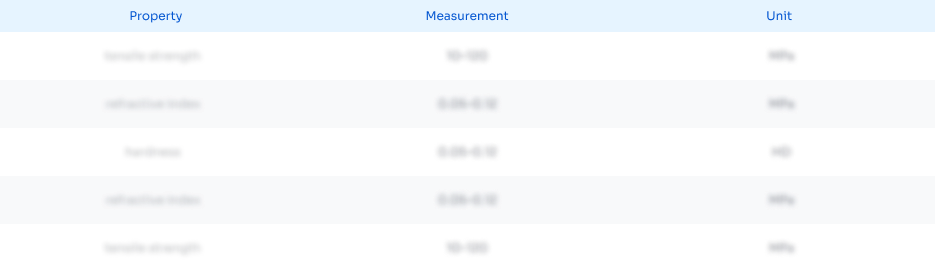
Abstract
Description
Claims
Application Information
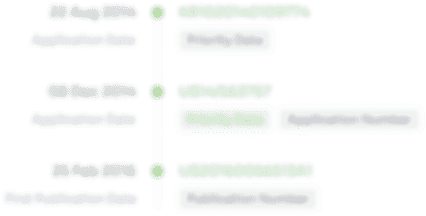
- R&D Engineer
- R&D Manager
- IP Professional
- Industry Leading Data Capabilities
- Powerful AI technology
- Patent DNA Extraction
Browse by: Latest US Patents, China's latest patents, Technical Efficacy Thesaurus, Application Domain, Technology Topic, Popular Technical Reports.
© 2024 PatSnap. All rights reserved.Legal|Privacy policy|Modern Slavery Act Transparency Statement|Sitemap|About US| Contact US: help@patsnap.com