Cross-modal subject correlation modeling method based on deep learning
A deep learning and correlation technology, applied in the field of cross-media correlation learning, can solve the problems of not considering the heterogeneity of images and texts well, and achieve the effects of high accuracy, efficiency promotion and strong adaptability
- Summary
- Abstract
- Description
- Claims
- Application Information
AI Technical Summary
Problems solved by technology
Method used
Image
Examples
Embodiment Construction
[0083] The cross-modal relevance calculation method for social images of the present invention will be described in detail below in conjunction with the accompanying drawings.
[0084] (1) Collection data object
[0085] Collect data objects, obtain images and image annotation data, and organize image annotation data that do not appear frequently or are useless in the entire data set. Generally, the obtained data set contains a lot of noise data, so it should be properly processed and filtered before using these data for feature extraction. For images, the obtained images are all in a uniform JPG format, and no conversion is required. For text annotation of images, the resulting image annotations contain a lot of meaningless words, such as words plus numbers without any meaning. Some images have as many as dozens of annotations. In order for the image annotations to describe the main information of the image well, those useless and meaningless annotations should be discarded...
PUM
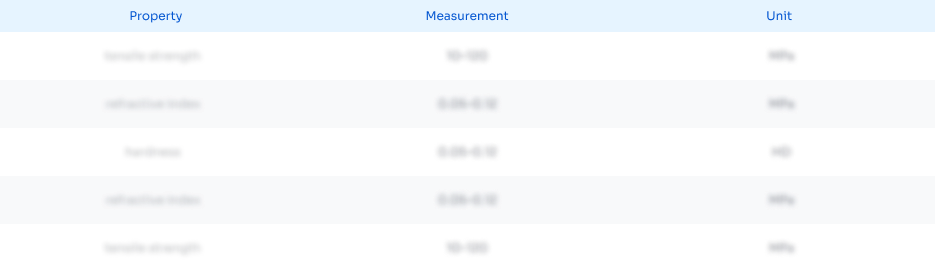
Abstract
Description
Claims
Application Information
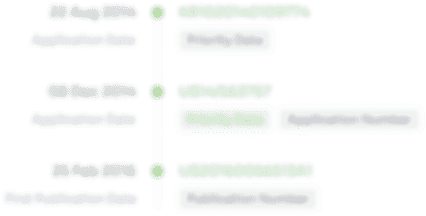
- R&D Engineer
- R&D Manager
- IP Professional
- Industry Leading Data Capabilities
- Powerful AI technology
- Patent DNA Extraction
Browse by: Latest US Patents, China's latest patents, Technical Efficacy Thesaurus, Application Domain, Technology Topic, Popular Technical Reports.
© 2024 PatSnap. All rights reserved.Legal|Privacy policy|Modern Slavery Act Transparency Statement|Sitemap|About US| Contact US: help@patsnap.com