Image super-resolution reconstruction method based on multi-task Gaussian process regression
A technique of Gaussian process regression and super-resolution reconstruction, which is applied in the field of image processing and can solve problems such as mapping functions that are insufficient to correctly describe GPR, large sample differences, and long spatial distances.
- Summary
- Abstract
- Description
- Claims
- Application Information
AI Technical Summary
Problems solved by technology
Method used
Image
Examples
Embodiment 1
[0092] The existing GPR super-resolution reconstruction algorithm is independent from task to task, and does not consider the relationship between tasks, so for each task, only a noise level σ is used to describe its characteristics, which is often inaccurate. The present invention introduces the idea of multi-task learning, not only using σ i To describe the noise level of each task, also use θ, The four parameters μ and ρ describe the commonality of tasks, and comprehensively utilize the commonality and differences between tasks to improve the accuracy of prediction.
[0093] The present invention proposes an image super-resolution reconstruction algorithm based on multi-task Gaussian process regression, the algorithm flow is as follows figure 2 As shown (wherein, the solid line arrow indicates the operation of the whole image, and the dotted line arrow indicates the operation for the image slice). image x 0 is the input image, Y 0 for X 0 The result of Gaussian lo...
PUM
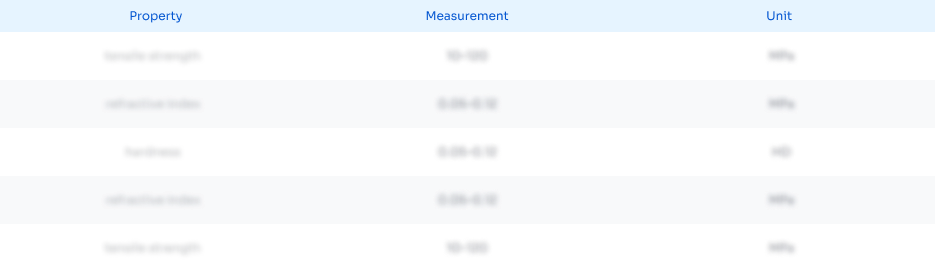
Abstract
Description
Claims
Application Information
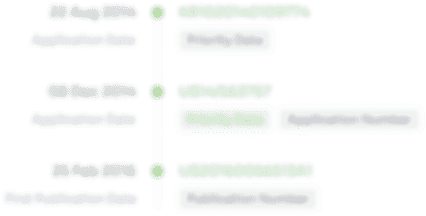
- R&D Engineer
- R&D Manager
- IP Professional
- Industry Leading Data Capabilities
- Powerful AI technology
- Patent DNA Extraction
Browse by: Latest US Patents, China's latest patents, Technical Efficacy Thesaurus, Application Domain, Technology Topic.
© 2024 PatSnap. All rights reserved.Legal|Privacy policy|Modern Slavery Act Transparency Statement|Sitemap