A large-scale semi-supervised feature selection method for high-resolution remote sensing images
A feature selection method and remote sensing image technology, applied in the direction of instruments, calculations, character and pattern recognition, etc., can solve the problems affecting the rationality of the selected features, achieve good adaptability, expand the application range, and reduce the effect of noise
- Summary
- Abstract
- Description
- Claims
- Application Information
AI Technical Summary
Problems solved by technology
Method used
Image
Examples
specific Embodiment approach 1
[0067] Specific implementation mode one: the following combination Figure 1 to Figure 6 Describe this embodiment, the large-scale semi-supervised feature selection method for high-resolution remote sensing images described in this embodiment, it includes the following steps:
[0068] Step 1: collect remote sensing image data, and preprocess the remote sensing image data; divide the preprocessed remote sensing image into n samples, perform feature extraction on each sample, and obtain sample data; and then extract each feature in the sample data After normalization processing, the normalized data X is obtained;
[0069] Step 2: Construct a probability distribution matrix {y based on the loss function and unlabeled samples for each feature in the normalized data X jk}’s metric function;
[0070] Step 3: cyclically optimize the three parameters of the measurement function obtained in step 2, and obtain the measurement value corresponding to the corresponding feature;
[0071]...
PUM
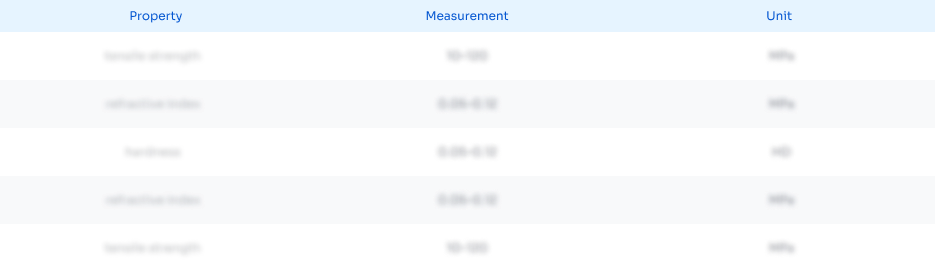
Abstract
Description
Claims
Application Information
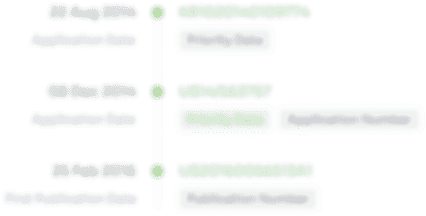
- R&D Engineer
- R&D Manager
- IP Professional
- Industry Leading Data Capabilities
- Powerful AI technology
- Patent DNA Extraction
Browse by: Latest US Patents, China's latest patents, Technical Efficacy Thesaurus, Application Domain, Technology Topic, Popular Technical Reports.
© 2024 PatSnap. All rights reserved.Legal|Privacy policy|Modern Slavery Act Transparency Statement|Sitemap|About US| Contact US: help@patsnap.com