A Large-Scale Data Mining Method Guaranteeing Quality Monotonicity
A large-scale data and monotonic technology, applied in the fields of electronic digital data processing, digital data information retrieval, special data processing applications, etc., can solve problems such as difficulty in mining result quality and resource constraints, approximate result quality monotonicity, etc.
- Summary
- Abstract
- Description
- Claims
- Application Information
AI Technical Summary
Problems solved by technology
Method used
Image
Examples
Embodiment Construction
[0046] The following examples describe the present invention in more detail.
[0047] The present invention proposes a large data mining method that guarantees quality monotonicity, the flow of the method is as follows figure 1 shown. The specific embodiment of the present invention is:
[0048] The first stage: perform operations such as data preprocessing on the data set, and represent the data as a form that can be processed by the mining part.
[0049] Step 1) Obtain the original iris data set (as shown in Table 1).
[0050] Step 2) Dimensionality reduction is performed on the data by principal component analysis method. Prevent the phenomenon of dimension disaster.
[0051] In this example, the iris data set contains information on 150 iris species, each of which is taken from one of three iris species: Setosa, Versicolour, and Virginica. The characteristics of each flower are described by the following 5 attributes:
[0052] (1) Sepal length (cm)
[0053] (2) Sepa...
PUM
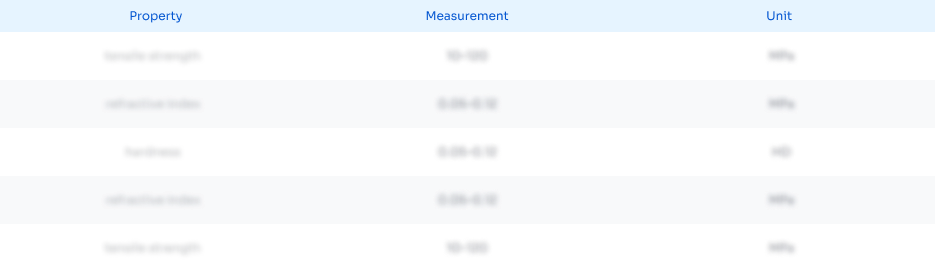
Abstract
Description
Claims
Application Information
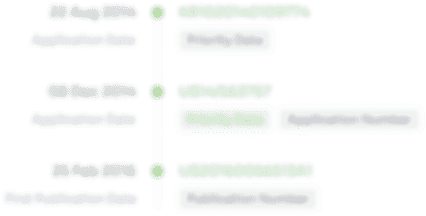
- R&D Engineer
- R&D Manager
- IP Professional
- Industry Leading Data Capabilities
- Powerful AI technology
- Patent DNA Extraction
Browse by: Latest US Patents, China's latest patents, Technical Efficacy Thesaurus, Application Domain, Technology Topic, Popular Technical Reports.
© 2024 PatSnap. All rights reserved.Legal|Privacy policy|Modern Slavery Act Transparency Statement|Sitemap|About US| Contact US: help@patsnap.com