From-bottom-to-top high-dimension-data causal network learning method
A learning method and causal network technology, applied in the field of data mining, can solve the problems of weak causal discovery of the global structure model and insufficient high-dimensional data expression ability of the local structure model
- Summary
- Abstract
- Description
- Claims
- Application Information
AI Technical Summary
Problems solved by technology
Method used
Image
Examples
Embodiment Construction
[0009] Corresponding to the three parts of the above method, the present invention is composed of three modules sequentially: a local causal structure generating module, a global directed acyclic graph topology sorting module based on causal strength measurement and a redundant causal relationship elimination module. The specific functions and implementation steps of these three modules are detailed as follows.
[0010] 1. Local causal structure generation module
[0011] Input: sample set D, variable set V, threshold α.
[0012] Output: causal relationship strength map G (including depicting the i-th variable and the j-th variable causal relationship v i → j measure of strength g ij and w ij ).
[0013] 1) Divide the variable set V into q disjoint sets of equal size, namely V 1 , V 2 ,...,V q . q recommended value Where m is the number of samples and n is the number of variables.
[0014] 2) Every two sets V i and V j (allowing i and j to be equal) form a subfie...
PUM
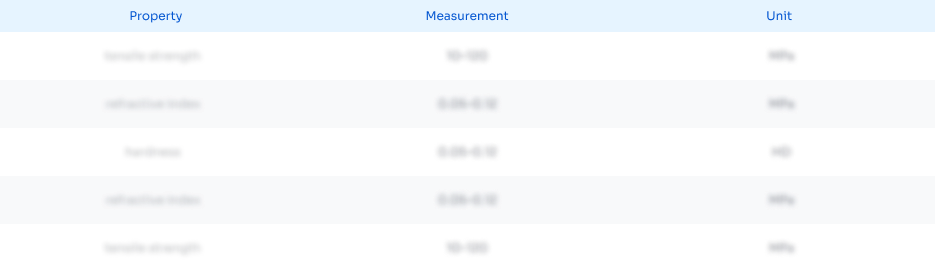
Abstract
Description
Claims
Application Information
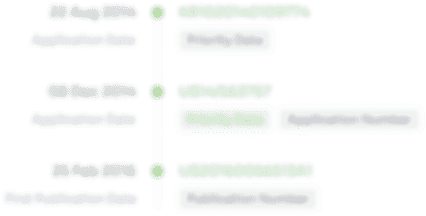
- Generate Ideas
- Intellectual Property
- Life Sciences
- Materials
- Tech Scout
- Unparalleled Data Quality
- Higher Quality Content
- 60% Fewer Hallucinations
Browse by: Latest US Patents, China's latest patents, Technical Efficacy Thesaurus, Application Domain, Technology Topic, Popular Technical Reports.
© 2025 PatSnap. All rights reserved.Legal|Privacy policy|Modern Slavery Act Transparency Statement|Sitemap|About US| Contact US: help@patsnap.com