Dialogue behavior recognition method based on deep neural network and conditional random field
A deep neural network, conditional random field technology, applied in speech recognition, character and pattern recognition, computer parts and other directions, can solve problems such as difficulty in recognizing intent, difficult to describe the dependency of label sequence, etc., and achieve the effect of good performance
- Summary
- Abstract
- Description
- Claims
- Application Information
AI Technical Summary
Problems solved by technology
Method used
Image
Examples
Embodiment Construction
[0048] The technical solutions of the present invention will be further described in detail below in conjunction with the accompanying drawings and specific embodiments.
[0049] The present invention proposes a complex sequence learning model based on multimodal deep learning and conditional random field (model structure such as figure 1 shown), and apply the model to solve Chinese dialogue act recognition. Using the multi-modal deep learning model to assist the setting of the state feature function in the conditional random field model not only makes up for the shortcomings of conditional random field and deep learning, but also can effectively deal with the challenges faced in the dialogue behavior recognition task.
[0050] Such as figure 1 Shown, a kind of dialog behavior recognition method based on deep neural network and conditional random field of the present invention comprises the following steps:
[0051] Step 1. Let the data set include Chinese spoken language co...
PUM
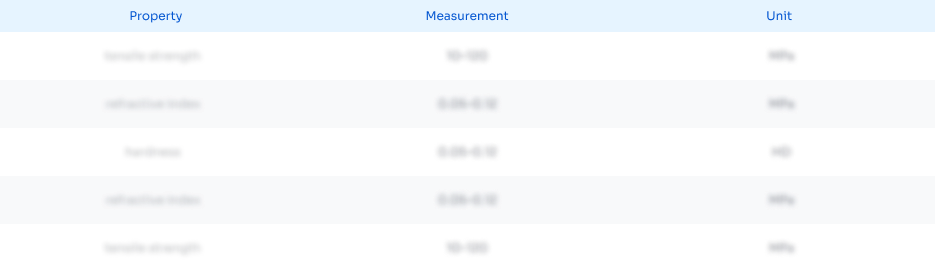
Abstract
Description
Claims
Application Information
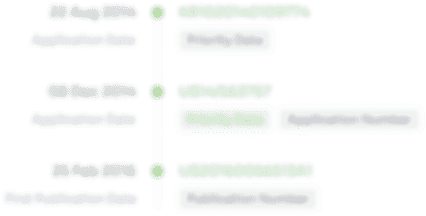
- R&D Engineer
- R&D Manager
- IP Professional
- Industry Leading Data Capabilities
- Powerful AI technology
- Patent DNA Extraction
Browse by: Latest US Patents, China's latest patents, Technical Efficacy Thesaurus, Application Domain, Technology Topic, Popular Technical Reports.
© 2024 PatSnap. All rights reserved.Legal|Privacy policy|Modern Slavery Act Transparency Statement|Sitemap|About US| Contact US: help@patsnap.com