Automatic labeling method for three-dimensional model component categories
A 3D model and automatic labeling technology, applied in image data processing, instruments, etc., can solve problems such as difficult segmentation, difficult to meet the labeling requirements of component parts, and inapplicability to different types of 3D models
- Summary
- Abstract
- Description
- Claims
- Application Information
AI Technical Summary
Problems solved by technology
Method used
Image
Examples
Embodiment
[0111] In this embodiment, a preprocessing process is performed on each 3D model in the training set and the 3D model of the target to be marked. Because this embodiment is an application for a 3D model, different component parts of the 3D model can only be distinguished using grayscale images. Firstly, through the feature extraction process, the unary features of each mesh patch are calculated, and the binary features of adjacent mesh patches, such as Figure 4b As shown, and then as Figure 4a Shown is the reflection symmetry plane detected by the symmetry screening step of the preprocessing process of a 3D model, so that the symmetry relationship obtained by this reflection symmetry transformation is used to delete redundant patches in the 3D model, so as to obtain the following Figure 4b Shown is the screened patch set, which includes binary features y and unary features x.
[0112] According to the example set model, the unary feature x and label of each patch in the p...
PUM
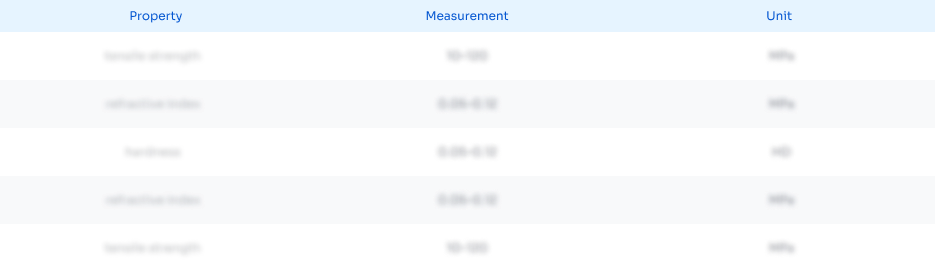
Abstract
Description
Claims
Application Information
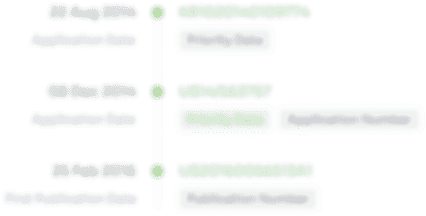
- R&D Engineer
- R&D Manager
- IP Professional
- Industry Leading Data Capabilities
- Powerful AI technology
- Patent DNA Extraction
Browse by: Latest US Patents, China's latest patents, Technical Efficacy Thesaurus, Application Domain, Technology Topic, Popular Technical Reports.
© 2024 PatSnap. All rights reserved.Legal|Privacy policy|Modern Slavery Act Transparency Statement|Sitemap|About US| Contact US: help@patsnap.com