Monte carlo characteristics dimension reduction method for small-sample hyperspectral image
A hyperspectral image and feature dimensionality reduction technology, applied in the fields of instruments, character and pattern recognition, computer parts, etc. The effect of increasing reliability, increasing complexity, increasing convenience
- Summary
- Abstract
- Description
- Claims
- Application Information
AI Technical Summary
Problems solved by technology
Method used
Image
Examples
Embodiment Construction
[0040] Concrete realization steps of the present invention are:
[0041] 1. Read in hyperspectral image sample data x i ∈ R N , where n is the number of sample pixels, each pixel has N band features, samples are divided into M categories, x i is a sample in the sample data set X, R N Represents the N-dimensional feature space, and the sample data set X is set to be divided into M subsets according to the category, Z={z 1 ,z 2 ,…,z M}, the number of samples in each category is {β i},i=1,2,...,M, where z i is the whole cluster of the i-th category in the sample X, and the sample label is the y i ∈{1,2,...,M}.
[0042] 2. Calculate the intra-class compactness and inter-class separation coefficients of the hyperspectral image sample data under the current sample label, where the intra-class compactness coefficient of the kth feature band of the sample is
[0043] Compactness Coefficient ( k ) = ...
PUM
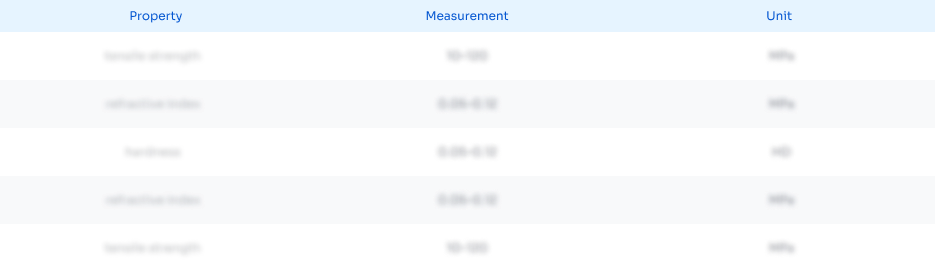
Abstract
Description
Claims
Application Information
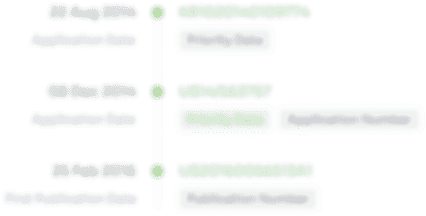
- R&D Engineer
- R&D Manager
- IP Professional
- Industry Leading Data Capabilities
- Powerful AI technology
- Patent DNA Extraction
Browse by: Latest US Patents, China's latest patents, Technical Efficacy Thesaurus, Application Domain, Technology Topic, Popular Technical Reports.
© 2024 PatSnap. All rights reserved.Legal|Privacy policy|Modern Slavery Act Transparency Statement|Sitemap|About US| Contact US: help@patsnap.com