Data stream effective clustering method based on tuple uncertainty
A clustering method and uncertainty technology, applied in the direction of electrical digital data processing, special data processing applications, instruments, etc., can solve problems that do not take into account the uncertainty of tuples, so as to improve the quality of clustering and fast processing speed Effect
- Summary
- Abstract
- Description
- Claims
- Application Information
AI Technical Summary
Problems solved by technology
Method used
Image
Examples
Embodiment Construction
[0032] The present invention will be further described below in conjunction with the drawings.
[0033] Reference figure 1 , An effective data stream clustering method based on tuple uncertainty, including the following steps:
[0034] 1) Initialization
[0035] Divide the memory into two areas: the main buffer BUF M And sub-buffer BUF V , Respectively store the microcluster information of the "normal" tuple and the "outlier" tuple. BUF M And BUF V Initialization is empty.
[0036] 2) Find the home cluster
[0037] For the data stream with uncertain existence of tuples S={ 1 , P 1 > , L, k , P k > , Each new tuple that arrives in L} Find the accepted clusters in the main buffer and the sub buffer respectively.
[0038] First, in the main candidate area is a new tuple Finding the home cluster includes the following steps:
[0039] Step1: If the main buffer is not full, the number of microclusters in the candidate area is less than the size of the main buffer n m (|BUF M |m ), then the...
PUM
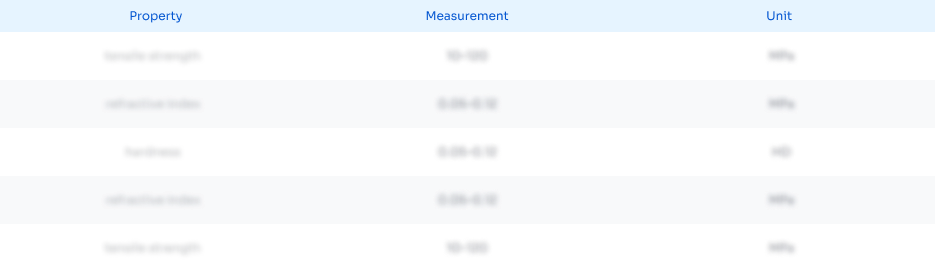
Abstract
Description
Claims
Application Information
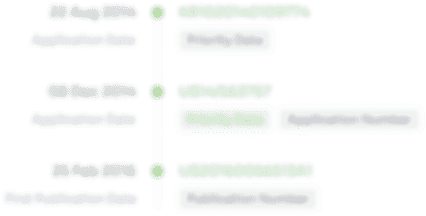
- R&D Engineer
- R&D Manager
- IP Professional
- Industry Leading Data Capabilities
- Powerful AI technology
- Patent DNA Extraction
Browse by: Latest US Patents, China's latest patents, Technical Efficacy Thesaurus, Application Domain, Technology Topic, Popular Technical Reports.
© 2024 PatSnap. All rights reserved.Legal|Privacy policy|Modern Slavery Act Transparency Statement|Sitemap|About US| Contact US: help@patsnap.com