Search-ranking-oriented sample selection method based on noise-adding active learning
A noise injection and active learning technology, applied in the field of machine learning, can solve the problems of affecting the final effect, low accuracy of the sorting model, and inaccurate estimation of expected loss, so as to reduce the cost and improve the performance of the model
- Summary
- Abstract
- Description
- Claims
- Application Information
AI Technical Summary
Problems solved by technology
Method used
Image
Examples
Embodiment
[0022] In this embodiment, for search ranking, the commercial search ranking data provided by Baidu is used for active learning sample selection. In this embodiment, the two most popular evaluation standards in the current information retrieval field, DCG10 and MAP (Mean Average Precision), are selected for effect evaluation, and experimental comparisons are made with existing representative sample selection techniques. The sample selection effect of the present invention can be fully tested. This embodiment includes the following steps:
[0023] In the first step, noise injection is performed for unlabeled samples.
[0024] Note that e∈[0, 1] is a d-dimensional unlabeled sample after 0-1 normalization, and the noise injection is expressed as follows:
[0025] e m =e+η
[0026] Among them, e m Indicates that m noise samples are generated after injecting noise from one sample, η is a d-dimensional vector, and obeys the Gaussian distribution p(η)~(μ, Σ), that is:
[0027] ...
PUM
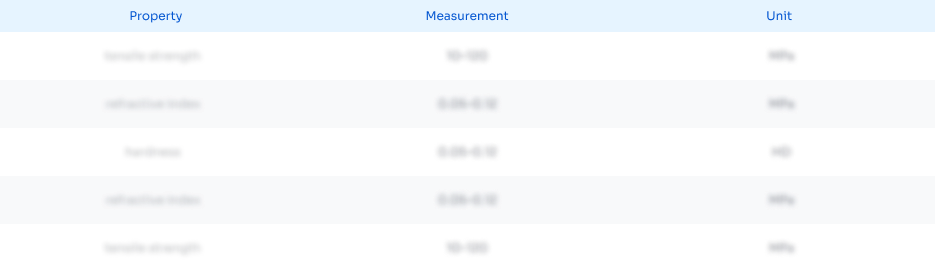
Abstract
Description
Claims
Application Information
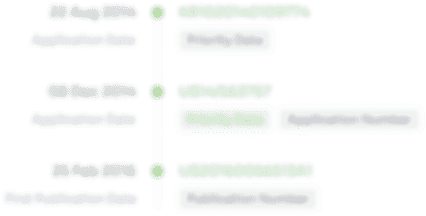
- R&D Engineer
- R&D Manager
- IP Professional
- Industry Leading Data Capabilities
- Powerful AI technology
- Patent DNA Extraction
Browse by: Latest US Patents, China's latest patents, Technical Efficacy Thesaurus, Application Domain, Technology Topic, Popular Technical Reports.
© 2024 PatSnap. All rights reserved.Legal|Privacy policy|Modern Slavery Act Transparency Statement|Sitemap|About US| Contact US: help@patsnap.com