Incremental learning-fused support vector machine multi-class classification method
A support vector machine and incremental learning technology, applied in the field of support vector machine multi-category classification, can solve problems such as hindering application, poor anti-interference ability, and high training data requirements, so as to achieve good generalization, save test time, and improve growth rate. The effect on the ability to quantify learning
- Summary
- Abstract
- Description
- Claims
- Application Information
AI Technical Summary
Problems solved by technology
Method used
Image
Examples
Embodiment 1
[0068] Example 1: Experimental results of BTMSVM without incremental learning training
[0069] Step 1: Pre-extract the training sample set to obtain a pre-extracted training sample set PTS consisting of 80 records;
[0070] The second step is to use PTS to train the iterative support vector machine to obtain the multi-class classification model M-SVM;
[0071] The third step is to perform binary tree processing on the M-SVM to obtain the support vector machine multi-class classification model BTMSVM based on the binary tree 0 , The training time is 10.92 seconds, 680 test samples are extracted to test the classification model, and the classification accuracy rate is 70.49%;
[0072] Step 4, add 20 training samples, merge them with 80 original training samples, total 100 records, and enter BTMSVM 0 For training, the training time is 23.75 seconds. Because the already trained classifier cannot be used (when the training sample is 80 hours), retraining is required, so the actual time sp...
Embodiment 2
[0078] Example 2: Experimental results of BTMSVM using incremental learning training
[0079] Step 1: Pre-extract the training sample set D to obtain the pre-extracted training sample set PTS consisting of 80 records;
[0080] The second step is to use PTS to train the iterative support vector machine to obtain the multi-class classification model M-SVM;
[0081] The third step is to perform binary tree processing on the M-SVM to obtain the support vector machine multi-class classification model BTMSVM based on the binary tree 0 , Training time 10.92 seconds;
[0082] The fourth step is to extract 680 test sample sets T through the multi-class classification model BTMSVM 0 Classification, the classification accuracy rate is 70.49%;
[0083] Step 5, add 20 records to the incremental sample set B 0 Input BTMSVM with 80 initial training samples PTS 0 Carry out incremental training, the incremental training time is 2.56 seconds, after the elimination rule of density method, a total of 5 rec...
PUM
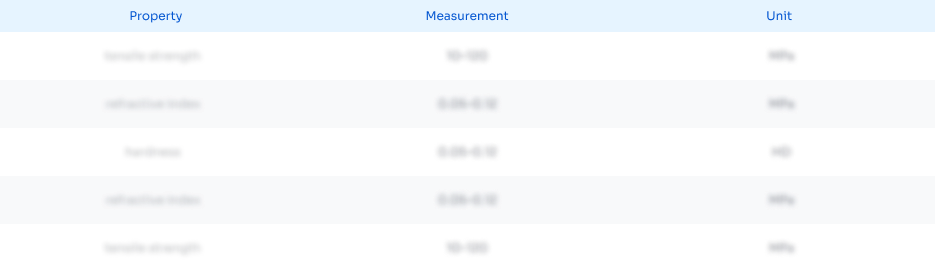
Abstract
Description
Claims
Application Information
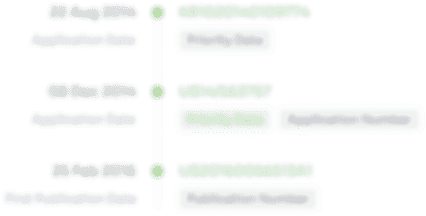
- R&D Engineer
- R&D Manager
- IP Professional
- Industry Leading Data Capabilities
- Powerful AI technology
- Patent DNA Extraction
Browse by: Latest US Patents, China's latest patents, Technical Efficacy Thesaurus, Application Domain, Technology Topic, Popular Technical Reports.
© 2024 PatSnap. All rights reserved.Legal|Privacy policy|Modern Slavery Act Transparency Statement|Sitemap|About US| Contact US: help@patsnap.com