Cascaded boosted predictive models
a predictive model and cascade technology, applied in the field of statistics-based machine learning, can solve the problems of limited use ability of existing approaches, existing methods that do not inherently take into account whether, and existing methods that do not distinguish between spontaneous observation and intentional elicitation features, so as to increase the likelihood of the rider having a positive experience, the effect of maximising the performance rating and increasing the likelihood of the driver receiving a higher performance rating scor
- Summary
- Abstract
- Description
- Claims
- Application Information
AI Technical Summary
Benefits of technology
Problems solved by technology
Method used
Image
Examples
Embodiment Construction
[0029]System Architecture
[0030]Turning now to the specifics of a system architecture for one application of a cascaded boosted predictive model system, FIG. 1 illustrates the system environment for an example transportation management system 130. Transportation management system 130 coordinates the transportation of persons and / or good / item for a user (“rider”) and a travel provider (“driver”) using a vehicle to provide the transportation. In this example embodiment, the transportation management system 130 includes, a trip management module 140, a trip matching module 145, a trip rating prediction module 150, a trip routing module 155, a trip monitoring module 165, a driver management module 160, a messaging module 170, and various data stores including a trip data store 180, a rider data store 182, a driver data store 184, a driver inventory data store 186, and a message data store 188. These modules and data stores utilize memory resources of the system 130 to store executable in...
PUM
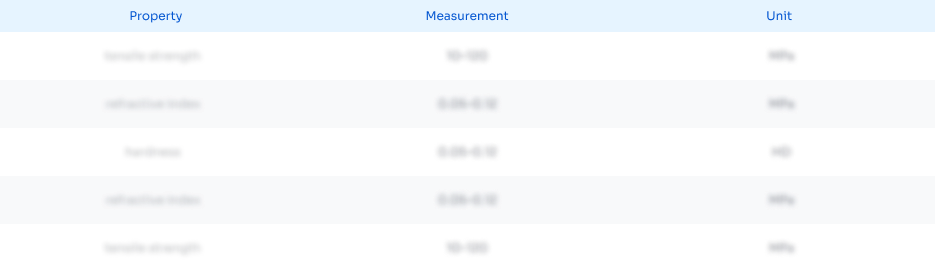
Abstract
Description
Claims
Application Information
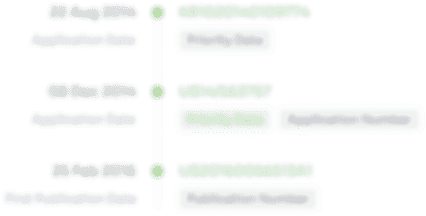
- R&D Engineer
- R&D Manager
- IP Professional
- Industry Leading Data Capabilities
- Powerful AI technology
- Patent DNA Extraction
Browse by: Latest US Patents, China's latest patents, Technical Efficacy Thesaurus, Application Domain, Technology Topic.
© 2024 PatSnap. All rights reserved.Legal|Privacy policy|Modern Slavery Act Transparency Statement|Sitemap