Electroencephalogram signal processing method based on synchronous compression wavelet transform and MLF-CNN
A MLF-CNN, EEG signal technology, applied in medical science, diagnosis, diagnostic recording/measurement, etc., can solve problems such as time resolution reduction, frequency resolution reduction, etc., to improve accuracy and time-frequency resolution. , the effect of preserving phase information
- Summary
- Abstract
- Description
- Claims
- Application Information
AI Technical Summary
Problems solved by technology
Method used
Image
Examples
Embodiment
[0055] In the model in this method, the CHB-MIT dataset was used as the EEG data for the study. The CHB-MIT dataset was collected at Boston Children's Hospital and contains a total of 24 EEG recordings of intractable epilepsy. All EEG data were sampled at a rate of 256Hz with a resolution of 16. Due to the missing temporal information of subject 24, we finally adopted the EEG data of the first 23 subjects. Fifteen minutes before the onset was selected as the pre-onset period, and the interictal data from at least 4 hours before the onset to 4 hours after the onset of the onset were selected to improve data quality and reduce interference.
[0056] Step 1. Data preprocessing
[0057] Denoise and filter the EEG signal data, and keep the 0-50Hz signal; remove the missing channels, keep FP1-F7, F7-T7, T7-P7, P7-O1, FP1-F3, F3-C3, C3-P3 ,P3-O1,FP2-F4,F4-C4,C4-P4,P4-O2,FP2-F8F8-T8,T8-P8,P8-O2,FZ-CZ,CZ-PZ channel signal. The time-domain data were divided into different segments, ...
PUM
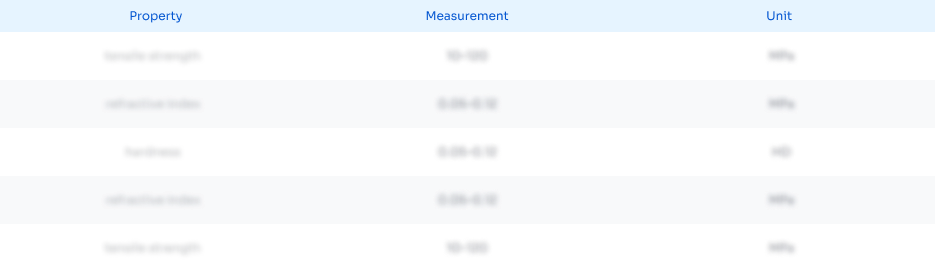
Abstract
Description
Claims
Application Information
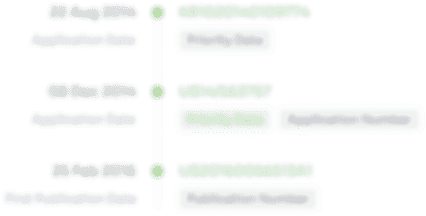
- R&D Engineer
- R&D Manager
- IP Professional
- Industry Leading Data Capabilities
- Powerful AI technology
- Patent DNA Extraction
Browse by: Latest US Patents, China's latest patents, Technical Efficacy Thesaurus, Application Domain, Technology Topic, Popular Technical Reports.
© 2024 PatSnap. All rights reserved.Legal|Privacy policy|Modern Slavery Act Transparency Statement|Sitemap|About US| Contact US: help@patsnap.com