Aero-engine rolling bearing fault diagnosis method based on twin network metric learning
An aero-engine and metric learning technology, applied in neural learning methods, biological neural network models, computer components, etc., can solve the problems of difficult to collect marked data, bearing mechanical failures that cannot be simulated by software, and difficult to collect valid data, etc. problems, achieving high fault diagnosis accuracy and high practical engineering application value
- Summary
- Abstract
- Description
- Claims
- Application Information
AI Technical Summary
Problems solved by technology
Method used
Image
Examples
Embodiment Construction
[0063] Now in conjunction with example, accompanying drawing the present invention is further described:
[0064] The fault diagnosis process of aero-engine bearings is as follows figure 1 As shown, the specific implementation steps of each step are described in detail below.
[0065] 1) Data preprocessing
[0066] short-time Fourier transform
[0067] In order to effectively extract the features of the collected original vibration signal, the original one-dimensional vibration signal is firstly subjected to short-time Fourier transform. The calculation method is as follows
[0068]
[0069] where x(m) is the input one-dimensional vibration signal, w(·) is the window function, n is the length of the window function, and the obtained X(n, ω) is a two-dimensional function defined by time and frequency. Using 2048 data points to form a vibration signal sample, after short-time Fourier transform, the obtained time-frequency feature map has a dimension of 2@257×103, where 2 r...
PUM
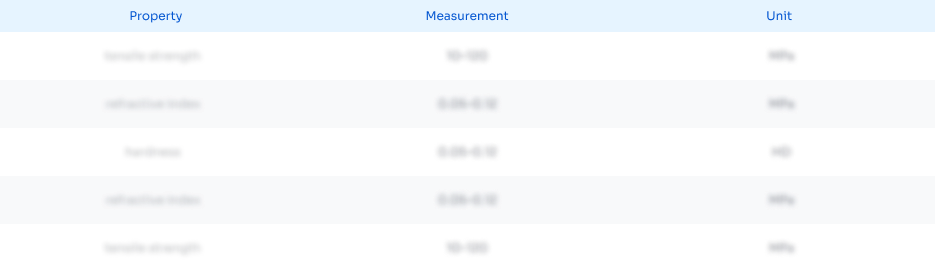
Abstract
Description
Claims
Application Information
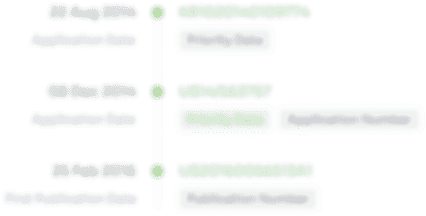
- R&D Engineer
- R&D Manager
- IP Professional
- Industry Leading Data Capabilities
- Powerful AI technology
- Patent DNA Extraction
Browse by: Latest US Patents, China's latest patents, Technical Efficacy Thesaurus, Application Domain, Technology Topic, Popular Technical Reports.
© 2024 PatSnap. All rights reserved.Legal|Privacy policy|Modern Slavery Act Transparency Statement|Sitemap|About US| Contact US: help@patsnap.com