Electrocardiosignal noise reduction method based on interpretable deep neural network
A deep neural network and electrocardiographic signal technology, applied in the field of electrocardiographic signal processing, can solve the problems of neural network lack of interpretability and robustness, and achieve the effect of improving interpretability and noise reduction ability
- Summary
- Abstract
- Description
- Claims
- Application Information
AI Technical Summary
Problems solved by technology
Method used
Image
Examples
Embodiment 1
[0066] In step a), ten clean signals of 103, 105, 111, 116, 122, 205, 213, 219, 223, and 230 in the MIT-BIH database are selected, and BW and EM in the noise pressure database in the MIT-BIH database are selected. , MA as noise data, by inputting the signal-to-noise ratio formula, the noise data with a signal-to-noise ratio of 1.25dB and 5dB is injected into the clean signal to obtain a noisy signal.
Embodiment 2
[0068] The training set samples divided in step b) are 480 segments, each segment has a length of 512, and the divided test set samples are 120 segments, each segment has a length of 512.
Embodiment 3
[0070] Step d) includes the following steps:
[0071] d-1) The first processing unit is sequentially composed of a convolutional layer with a channel number of 64, a convolution kernel size of 1×3, and a LeakyReLU activation function. After the training set is input to the first processing unit, the output channel number is 64-dimensional. characteristic signal T 1 ;
[0072] d-2) The second processing unit is sequentially composed of a convolutional layer with a channel number of 64, a convolution kernel size of 1×5, and a LeakyReLU activation function. After the training set is input to the second processing unit, the output channel number is 64-dimensional. characteristic signal T 2 ;
[0073] d-3) The third processing unit is sequentially composed of a convolutional layer with 64 channels, a convolution kernel size of 1×7, and a LeakyReLU activation function. After the training set is input to the third processing unit, the output channel number is 64-dimensional. char...
PUM
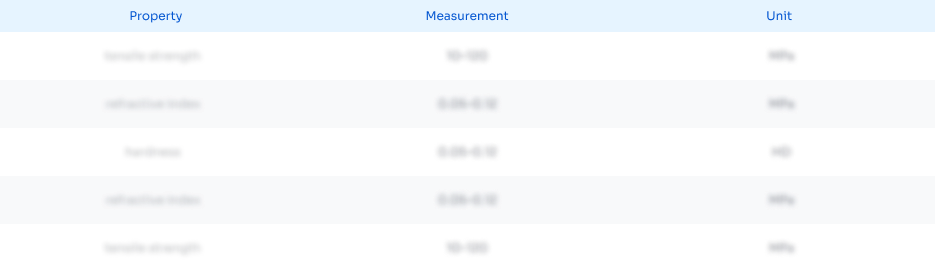
Abstract
Description
Claims
Application Information
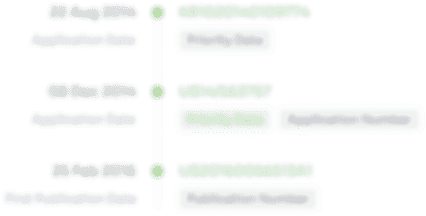
- R&D Engineer
- R&D Manager
- IP Professional
- Industry Leading Data Capabilities
- Powerful AI technology
- Patent DNA Extraction
Browse by: Latest US Patents, China's latest patents, Technical Efficacy Thesaurus, Application Domain, Technology Topic, Popular Technical Reports.
© 2024 PatSnap. All rights reserved.Legal|Privacy policy|Modern Slavery Act Transparency Statement|Sitemap|About US| Contact US: help@patsnap.com