Handwritten numeral generation method based on double-discriminator weighted hybrid generative adversarial network
A dual discriminator and discriminator technology, applied in biological neural network models, neural learning methods, instruments, etc., can solve problems such as inability to use handwritten digit generation methods, mode collapse, gradient disappearance, etc., to solve the gradient disappearance. Issues, effects to fix modal crash issues
- Summary
- Abstract
- Description
- Claims
- Application Information
AI Technical Summary
Problems solved by technology
Method used
Image
Examples
Embodiment 1
[0126] like figure 1 As shown, the handwritten digit generation method based on the dual discriminator weighted hybrid generative adversarial network of the present invention, the method comprises the following steps:
[0127] Step 1: Obtain the real handwritten digital image of the target user;
[0128] Step 2: Input the real handwritten digital image of the target user into the pre-trained dual discriminator weighted hybrid generative adversarial network (D2WMGAN network); wherein, the training process of the dual discriminator weighted hybrid generative adversarial network (D2WMGAN network) is as follows: :
[0129] Step 201, using the MNIST data set as a training sample;
[0130] The MNIST dataset consists of handwritten digits from 250 different people, 50% of whom are high school students and 50% from the Census Bureau staff. The MNIST dataset contains 70,000 pictures of handwritten digits, of which 60,000 are The training set, 10,000 as the test set, is divided into ...
Embodiment 2
[0179] The difference between this embodiment and Embodiment 1 is that the training process of the dual discriminator weighted hybrid generative adversarial network (D2WMGAN network) described in step 2 further includes step 205, which is to perform the training of the double discriminator weighted hybrid generative adversarial network (D2WMGAN network). Network) to carry out theoretical analysis and verification, and verify that under the optimal discriminator, the generator generates real handwritten digital data by minimizing the KL divergence and the reverse KL divergence between the generated data and the real data.
[0180] In this embodiment, in step 205, the trained dual discriminator weighted hybrid generative adversarial network (D2WMGAN network) is theoretically analyzed and verified to verify that under the optimal discriminator, by minimizing the difference between the generated data and the real data KL divergence and reverse KL divergence, the specific process fo...
PUM
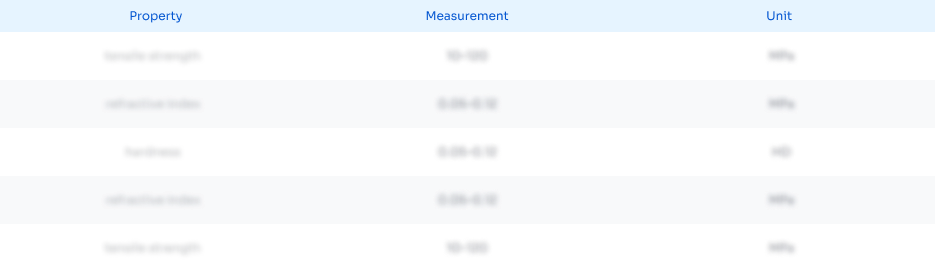
Abstract
Description
Claims
Application Information
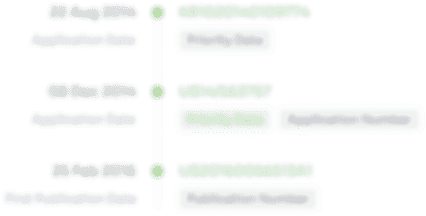
- R&D Engineer
- R&D Manager
- IP Professional
- Industry Leading Data Capabilities
- Powerful AI technology
- Patent DNA Extraction
Browse by: Latest US Patents, China's latest patents, Technical Efficacy Thesaurus, Application Domain, Technology Topic, Popular Technical Reports.
© 2024 PatSnap. All rights reserved.Legal|Privacy policy|Modern Slavery Act Transparency Statement|Sitemap|About US| Contact US: help@patsnap.com