Electrocardiogram diagnosis method and device based on artificial rule enhanced neural network
A technology of neural network and diagnosis method, applied in the field of electrocardiogram diagnosis based on artificial rule-enhanced neural network, can solve the problems of complex waveform superposition, variable signal superposition, difficult diagnosis, etc., to improve the diagnosis accuracy, reduce the use threshold, network interpretation strong effect
- Summary
- Abstract
- Description
- Claims
- Application Information
AI Technical Summary
Problems solved by technology
Method used
Image
Examples
Embodiment Construction
[0040] The present invention will be further described in detail below with reference to the accompanying drawings and embodiments. It should be pointed out that the following embodiments are intended to facilitate the understanding of the present invention, but do not have any limiting effect on it.
[0041] like figure 1 As shown, the multi-lead ECG signal diagnosis model of artificial rule-enhanced neural network includes a training process and a testing process. Specifically include the following steps:
[0042] data collection:
[0043] In this example, the data of 200 cases of on-board patients were collected from Run Run Shaw Hospital affiliated to Zhejiang University School of Medicine.
[0044] algorithm design:
[0045] The present invention uses artificial rules to enhance the multi-lead electrocardiogram signal diagnosis model of neural network (such as figure 2 ). The neural network basic network used in this case uses ResNet. In fact, the specific operation i...
PUM
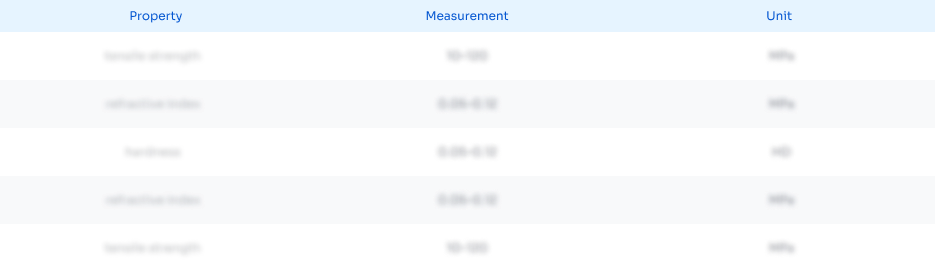
Abstract
Description
Claims
Application Information
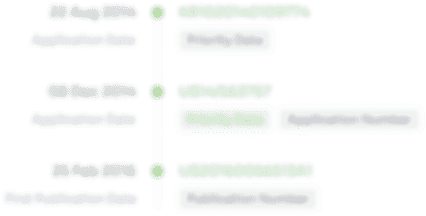
- R&D Engineer
- R&D Manager
- IP Professional
- Industry Leading Data Capabilities
- Powerful AI technology
- Patent DNA Extraction
Browse by: Latest US Patents, China's latest patents, Technical Efficacy Thesaurus, Application Domain, Technology Topic, Popular Technical Reports.
© 2024 PatSnap. All rights reserved.Legal|Privacy policy|Modern Slavery Act Transparency Statement|Sitemap|About US| Contact US: help@patsnap.com