Low bit width bias cross-border processing method in quantitative reasoning process
A technology of reasoning process and processing method, which is applied in the field of low-bit-width bias out-of-bounds processing in the quantitative inference process, and can solve problems such as affecting the quantitative inference accuracy of models, model inference errors, and bias out-of-bounds.
- Summary
- Abstract
- Description
- Claims
- Application Information
AI Technical Summary
Problems solved by technology
Method used
Image
Examples
Embodiment Construction
[0033] In order to understand the technical content and advantages of the present invention more clearly, the present invention will now be further described in detail with reference to the accompanying drawings.
[0034] This application belongs to the processing method of deep neural network based on low-bit (4bit, 5bit) training and prone to bias out-of-bounds in the forward reasoning process. The output results on the inference library are consistent with the output results of the network training framework. It is a processing method to prevent bias out-of-bounds in low-bit quantization bit width and ensure the correct forward inference of the model.
[0035] Based on the low-bit quantization operation process to ensure the accuracy of model inference and reduce the loss of accuracy in the process of model inference, the gamma value of the batchnorm channel is processed separately to avoid the problem of bias outliers in the batchnorm during the weight merging process. The...
PUM
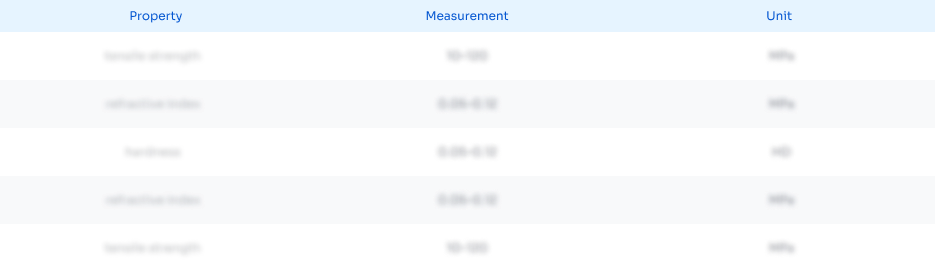
Abstract
Description
Claims
Application Information
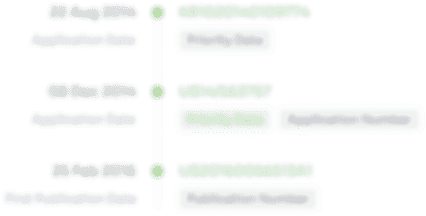
- R&D Engineer
- R&D Manager
- IP Professional
- Industry Leading Data Capabilities
- Powerful AI technology
- Patent DNA Extraction
Browse by: Latest US Patents, China's latest patents, Technical Efficacy Thesaurus, Application Domain, Technology Topic, Popular Technical Reports.
© 2024 PatSnap. All rights reserved.Legal|Privacy policy|Modern Slavery Act Transparency Statement|Sitemap|About US| Contact US: help@patsnap.com