Lithium battery temperature field online modeling method and system
A modeling method and temperature field technology, applied in the field of lithium battery temperature field online modeling method and system, can solve problems such as aggravating the generation of ill-conditioned matrix inversion and algorithm instability, and achieve the effect of ensuring timeliness and accurate prediction
- Summary
- Abstract
- Description
- Claims
- Application Information
AI Technical Summary
Problems solved by technology
Method used
Image
Examples
Embodiment 1
[0102] like figure 1 As shown in the figure, an online modeling method for a temperature field of a lithium battery includes the following steps;
[0103] S1. Use the KL method to separate the space-time data in space and time, and obtain the space basis function and time coefficient respectively;
[0104] S2. Use the width learning network to train the time coefficient to obtain an offline model of the temperature field of the lithium battery;
[0105] S3. Use the incremental learning algorithm of the width learning network to update the network weight of the offline model. When the set weight value is reached, stop the update to obtain the online model of the lithium battery temperature field;
[0106] S4, reconstruct the prediction time coefficient in the online model and the space basis function obtained in step S1 to obtain a prediction model;
[0107] S5. Input the temperature field data of the lithium battery to be predicted into the prediction model, and the predicti...
Embodiment 2
[0189] like figure 2 As shown, this embodiment is similar to Embodiment 1, the difference is that this embodiment provides an online modeling system for lithium battery temperature field based on the principle of the method for the method in Embodiment 1, and the system includes a processor , and a separation module, a training module, an update module, and a reconstruction module that are communicatively connected to the processor;
[0190] The separation module uses the KL method to separate the space-time data, obtains the space basis function and the time coefficient, and uploads them to the processor, and the processor sends the time coefficient to the training module;
[0191] The training module uses the width learning network to train the time coefficient, obtains the offline model of the lithium battery temperature field, and sends it to the update module;
[0192] The update module uses the incremental learning algorithm of the width learning network to update the ...
Embodiment 3
[0198] Taking the thermal process of a 32Ah NCM ternary lithium battery as an example, the embodiments of the present invention will be explained.
[0199] There are 32 thermal sensors evenly distributed on the surface of the lithium battery. In order to fully excite the battery temperature, the input current and the generated operating voltage are used as the input signal u(t)=[I(t), U(t)], and I(t) is Input current; U(t) is the working voltage. In the case of the sample interval Δt=1s, the data of 1370s since the discharge is used, a total of 1370 groups, each group contains 32 temperature data collected by the thermal sensor.
[0200] In the initial stage, using the KL method to separate the spatiotemporal data for the temperature data y(x, t), the spatiotemporal data can be separated into spatial basis functions containing spatial information and time-related corresponding time coefficients.
[0201] When using the snapshot method to find the spatial basis function, the f...
PUM
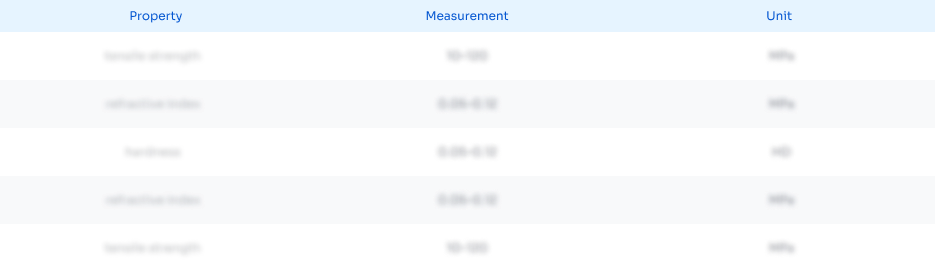
Abstract
Description
Claims
Application Information
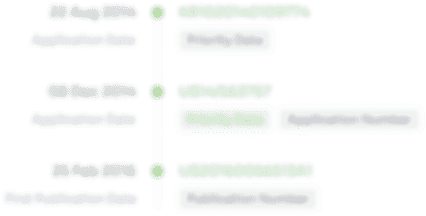
- R&D Engineer
- R&D Manager
- IP Professional
- Industry Leading Data Capabilities
- Powerful AI technology
- Patent DNA Extraction
Browse by: Latest US Patents, China's latest patents, Technical Efficacy Thesaurus, Application Domain, Technology Topic, Popular Technical Reports.
© 2024 PatSnap. All rights reserved.Legal|Privacy policy|Modern Slavery Act Transparency Statement|Sitemap|About US| Contact US: help@patsnap.com