Medical image segmentation method based on boosting-unet segmentation network
A medical imaging and network technology, applied in neural learning methods, image analysis, biological neural network models, etc., can solve the problem of low image blur resistance, improve learning ability, improve performance, and reduce the number of effects
- Summary
- Abstract
- Description
- Claims
- Application Information
AI Technical Summary
Problems solved by technology
Method used
Image
Examples
Embodiment 1
[0074] The dataset used in this example comes from a pancreatic cancer-related CT slice image dataset provided by a hospital, wherein the CT slice image dataset includes the CT slice image data of the arterial phase and the portal venous phase, and contains CT slice images of 69 cases in total. Slice images, a total of 27184 CT slice images, occupying a total of 48GB space, this example uses the constructed , layer total segmentation network, such as image 3 As shown in the figure, the segmentation of the pancreas and its pancreatic cancer in CT slice images includes the following steps:
[0075] S1: Obtain the CT slice image data set, truncate and normalize the CT slice image data set: according to the grayscale interval of the CT slice image data , assuming a certain pixel value in the original image data , after truncation, the pixel value is , then after normalization, the pixel value is , perform the same operation on all CT slice image data, take the processe...
Embodiment 2
[0084] The data set used in this example is the same as that in Example 1, and this example uses the constructed of layer total segmentation network, such as Figure 4 As shown in the figure, the segmentation of the pancreas and its pancreatic cancer in CT slice images includes the following steps:
[0085] S1: Obtain the CT slice image data set, truncate and normalize the CT slice image data set: according to the grayscale interval of the CT slice image data , assuming a certain pixel value in the original image data , after truncation, the pixel value is , then after normalization, the pixel value is , perform the same operation on all CT slice image data, take the processed CT slice image data as input, and also need to back up a copy of CT slice image data as the marked and segmented CT slice image data; The annotated and segmented CT slice image dataset is divided into training set and validation set.
[0086] S2: Build contains The total segmentation networ...
Embodiment 3
[0095] The data set used in this example is the same as that in Example 1, and this example uses the constructed layer total segmentation network, such as Figure 5 As shown in the figure, the segmentation of the pancreas and its pancreatic cancer in CT slice images includes the following steps:
[0096] S1: Obtain the CT slice image data set, truncate and normalize the CT slice image data set: according to the grayscale interval of the CT slice image data , assuming a certain pixel value in the original image data , after truncation, the pixel value is , then after normalization, the pixel value is , perform the same operation on all CT slice image data, take the processed CT slice image data as input, and also need to back up a copy of CT slice image data as the marked and segmented CT slice image data; The annotated and segmented CT slice image dataset is divided into training set and validation set.
[0097] S2: Build contains The total segmentation network of ...
PUM
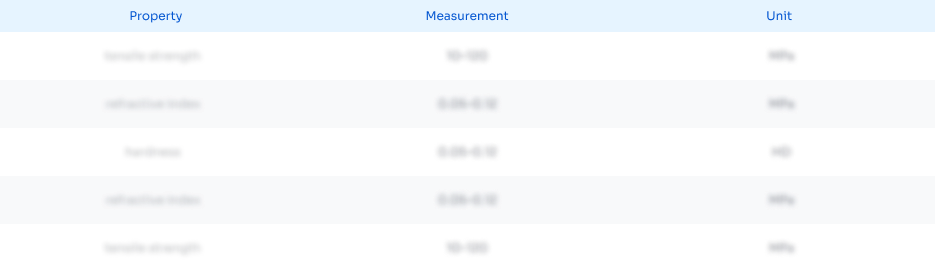
Abstract
Description
Claims
Application Information
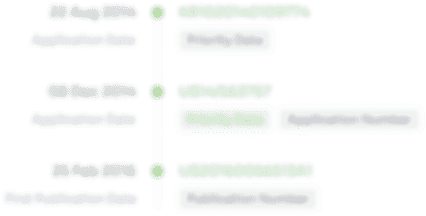
- R&D Engineer
- R&D Manager
- IP Professional
- Industry Leading Data Capabilities
- Powerful AI technology
- Patent DNA Extraction
Browse by: Latest US Patents, China's latest patents, Technical Efficacy Thesaurus, Application Domain, Technology Topic, Popular Technical Reports.
© 2024 PatSnap. All rights reserved.Legal|Privacy policy|Modern Slavery Act Transparency Statement|Sitemap|About US| Contact US: help@patsnap.com