Post-stroke rehabilitation evaluation deep learning model construction method based on brain muscle network graph theory characteristics
A construction method and deep learning technology, applied in the intersection of neurophysiology and machine learning, which can solve problems such as the lack of application of the brain-muscle coupled bidirectional pathway, the inability to fully represent the closed-loop evolution process of the bidirectional pathway, and the lack of consideration of the local nature of the lesion site.
- Summary
- Abstract
- Description
- Claims
- Application Information
AI Technical Summary
Problems solved by technology
Method used
Image
Examples
Embodiment Construction
[0026] The following describes several preferred embodiments of the present invention with reference to the accompanying drawings, so as to make its technical content clearer and easier to understand. The present invention can be embodied in many different forms of embodiments, and the protection scope of the present invention is not limited to the embodiments mentioned herein.
[0027] The invention provides a deep learning model construction method for post-stroke rehabilitation assessment based on the characteristics of brain muscle network graph theory, according to the following steps: figure 1 The method steps shown are implemented. The invention mines the topological pattern characteristics that can characterize the current recovery period of the patient, establishes a multi-layer feedforward neural network model under the constraint of a specific motion paradigm, dynamically evaluates the motion function of the affected limb of the patient, and benchmarks the clinical ...
PUM
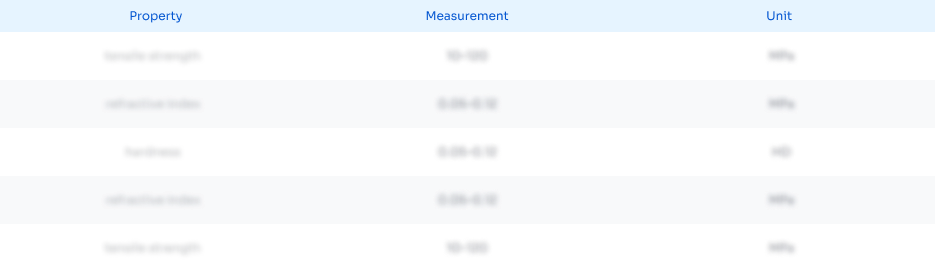
Abstract
Description
Claims
Application Information
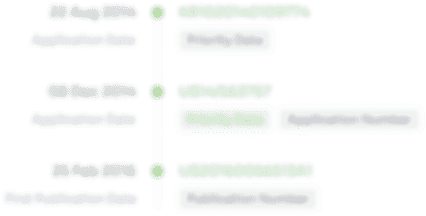
- R&D Engineer
- R&D Manager
- IP Professional
- Industry Leading Data Capabilities
- Powerful AI technology
- Patent DNA Extraction
Browse by: Latest US Patents, China's latest patents, Technical Efficacy Thesaurus, Application Domain, Technology Topic, Popular Technical Reports.
© 2024 PatSnap. All rights reserved.Legal|Privacy policy|Modern Slavery Act Transparency Statement|Sitemap|About US| Contact US: help@patsnap.com