Picture table line extraction model construction method and picture table extraction method
A technology of construction method and extraction method, applied in the field of graphic extraction, can solve cumbersome and complex problems, achieve the effect of good recognition effect, reduce errors, and improve the accuracy of the model
- Summary
- Abstract
- Description
- Claims
- Application Information
AI Technical Summary
Problems solved by technology
Method used
Image
Examples
Embodiment 1
[0049] This example builds an image table extraction model, including the following steps:
[0050] Step 1: Select training data; build a dataset.
[0051]Traditional region-based semantic segmentation methods first extract free-form regions from an image and describe their features, then classify them based on regions, and finally convert region-based predictions to pixel-level predictions using pixel-inclusive classification categories. The highest scoring regions are used to label pixels. This method needs to generate a large number of candidate regions, which takes a lot of time and memory space. However, using this method to mark the line segment area in the picture will cause the pixels of the line segment area and the line segment edge area to overlap, the marking effect is not good, and the marking is too cumbersome and complicated.
[0052] In this example, the image containing the table is analyzed, each table line is marked with a line segment of a preset width, an...
Embodiment 2
[0065] This example is based on the model constructed in Example 1, and describes the selection of its loss function, as follows:
[0066] (1) The U-Net and U-Net++ models are both image semantic segmentation models, which are pixel-level classification tasks. The most commonly used loss function for image semantic segmentation tasks is the cross entropy loss function, which is calculated as follows:
[0067] row loss=-∑y true log(y pred )
[0068] As you can see from this cross-entropy loss function, when y true is 0, that is, the loss of pixels not marked as horizontal lines in the input is 0, only when y true When it is 1, that is, the loss value exists only when the pixels in the input are marked as horizontal lines, so if this cross entropy loss function is used for calculation, the loss of most pixels will be discarded, resulting in poor model training effect. good.
[0069] (2) In order to solve the above problems, a binary cross-entropy loss function, namely BCE L...
Embodiment 3
[0078] This example provides a method for extracting picture table information. The process of extracting picture table information based on deep learning includes the following steps:
[0079] Step 1: Perform layout analysis on the picture and extract the table area.
[0080] For a picture, it may contain one or more tables, or it may not contain tables, so it is necessary to find the table area in the picture before extracting the table lines and reconstructing the table.
[0081] Step 2: Build any one of the image table extraction models in Embodiment 1, apply it to table extraction for each table area, determine the category of each pixel in the area, and determine table lines. The specific model selection has been specifically described in Embodiment 1, and will not be repeated here.
[0082] This example uses the integrated model based on U-Net and U-Net++, and the output probability of U-Net and U-Net++ is directly added or weighted as the final output of the model. W...
PUM
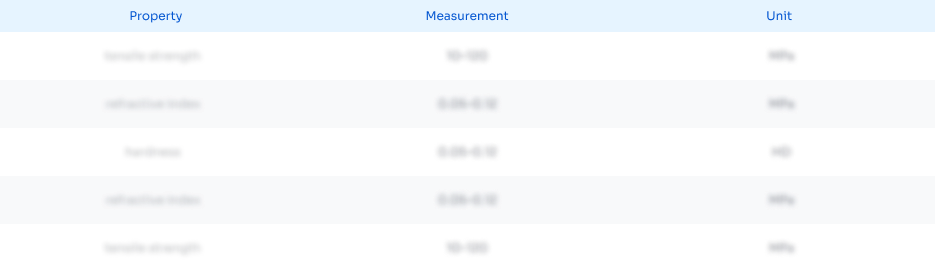
Abstract
Description
Claims
Application Information
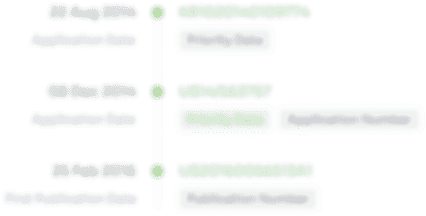
- R&D Engineer
- R&D Manager
- IP Professional
- Industry Leading Data Capabilities
- Powerful AI technology
- Patent DNA Extraction
Browse by: Latest US Patents, China's latest patents, Technical Efficacy Thesaurus, Application Domain, Technology Topic, Popular Technical Reports.
© 2024 PatSnap. All rights reserved.Legal|Privacy policy|Modern Slavery Act Transparency Statement|Sitemap|About US| Contact US: help@patsnap.com