Discrete manufacturing workshop digital twin model adaptive dynamic updating method
A discrete manufacturing workshop and model adaptive technology, applied in the field of workshop digitalization, can solve problems such as inaccurate performance models and twin models that cannot be dynamically corrected, and achieve the effects of simple theoretical methods, meeting the requirements of adaptive update, and improving computing efficiency
- Summary
- Abstract
- Description
- Claims
- Application Information
AI Technical Summary
Problems solved by technology
Method used
Image
Examples
Embodiment Construction
[0062] The present invention will be further described below in conjunction with the accompanying drawings. Apparently, the described embodiments are some, but not all, embodiments of the present invention. Based on the embodiments of the present invention, all other embodiments obtained by persons of ordinary skill in the art without making creative efforts belong to the protection scope of the present invention.
[0063] The invention provides a method for adaptive dynamic updating of a digital twin model of a discrete manufacturing workshop, which specifically includes the following steps:
[0064] Step S1, the Internet of Things sensing equipment deployed in the digital twin manufacturing workshop collects production data of multiple continuous production processes in time sequence, and selects a feature data set; the feature data set includes order task data, completed task data at the current moment, and real-time production data. Status data and forecast time; data col...
PUM
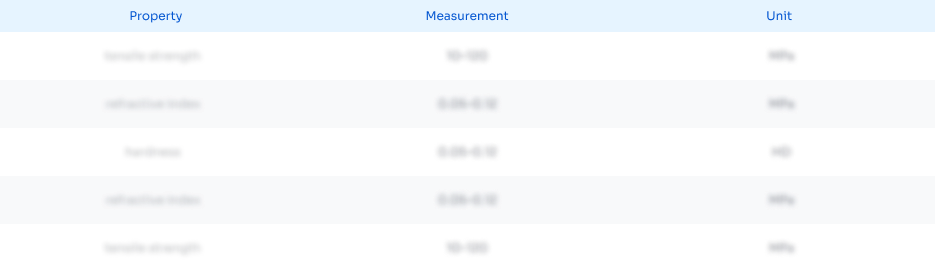
Abstract
Description
Claims
Application Information
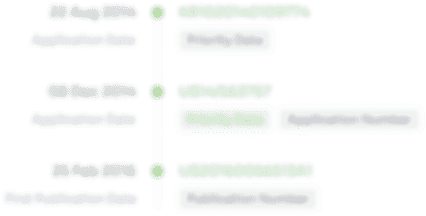
- R&D
- Intellectual Property
- Life Sciences
- Materials
- Tech Scout
- Unparalleled Data Quality
- Higher Quality Content
- 60% Fewer Hallucinations
Browse by: Latest US Patents, China's latest patents, Technical Efficacy Thesaurus, Application Domain, Technology Topic, Popular Technical Reports.
© 2025 PatSnap. All rights reserved.Legal|Privacy policy|Modern Slavery Act Transparency Statement|Sitemap|About US| Contact US: help@patsnap.com