Vehicle-mounted high-definition map data source selection method and device
A technology of map data and data sources, applied in the field of deep learning, can solve the problems of frequent RTT updates, reduced throughput, inefficient data transmission, etc., and achieve the effect of avoiding data source switching and reducing throughput
- Summary
- Abstract
- Description
- Claims
- Application Information
AI Technical Summary
Problems solved by technology
Method used
Image
Examples
Embodiment Construction
[0067] Embodiments of the present invention are described in detail below, and examples of the embodiments are shown in the drawings, wherein the same or similar reference numerals denote the same or similar elements or elements having the same or similar functions throughout. The embodiments described below by referring to the figures are exemplary and are intended to explain the present invention and should not be construed as limiting the present invention.
[0068] The present invention proposes a method for selecting a data source of a vehicle-mounted high-definition map, which uses a deep reinforcement learning algorithm to learn and train a neural network based on collected empirical data to generate a selection strategy for data source selection. The map selection network consists of four main parts, namely state information, action, policy and reward. In order to simulate the dynamics of the vehicle scene, the state information of the data source is represented by veh...
PUM
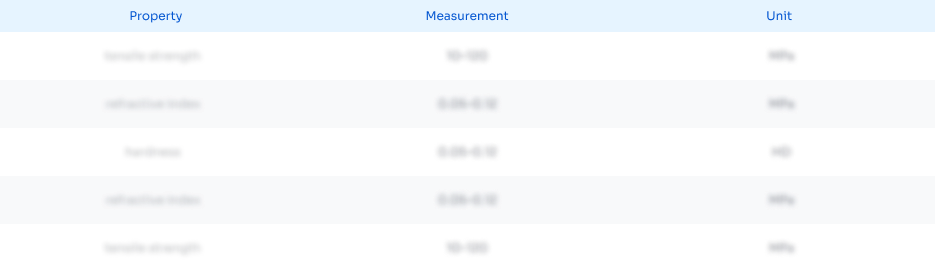
Abstract
Description
Claims
Application Information
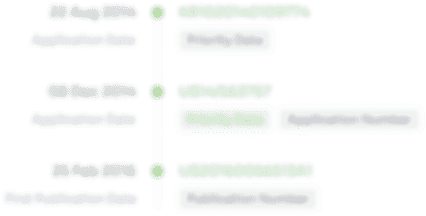
- R&D Engineer
- R&D Manager
- IP Professional
- Industry Leading Data Capabilities
- Powerful AI technology
- Patent DNA Extraction
Browse by: Latest US Patents, China's latest patents, Technical Efficacy Thesaurus, Application Domain, Technology Topic, Popular Technical Reports.
© 2024 PatSnap. All rights reserved.Legal|Privacy policy|Modern Slavery Act Transparency Statement|Sitemap|About US| Contact US: help@patsnap.com