Improved gesture image feature extraction method based on DenseNet network
An image feature extraction and gesture technology, applied in neural learning methods, biological feature recognition, biological neural network models, etc., can solve problems such as difficult to accurately identify information and redundancy, and achieve suppression of overfitting and reduction of feature information redundancy , the effect of speeding up network training
- Summary
- Abstract
- Description
- Claims
- Application Information
AI Technical Summary
Problems solved by technology
Method used
Image
Examples
Embodiment Construction
[0056] The present invention will be further described below in conjunction with the accompanying drawings and embodiments.
[0057] The technical scheme flow chart of the present invention is as figure 1 shown.
[0058] Data set of the present invention adopts ASL (American sign language) open source sign language data set, and its part data are as figure 2 shown. Contains gesture images of different gesture target proportions, different angles, different lighting and different background environments, including 28 gesture categories and 1 non-gesture category, a total of 29 classification categories.
[0059] 1) Normalize the size of the original gesture image to a 224×224×3 RGB image, then normalize the original gesture image, and map the original gesture image from an integer between 0 to 255 to a float between 0 and 1 The points are used as input to the neural network.
[0060] 2) Input the standardized gesture image figure 1 In the downsampling network shown, the d...
PUM
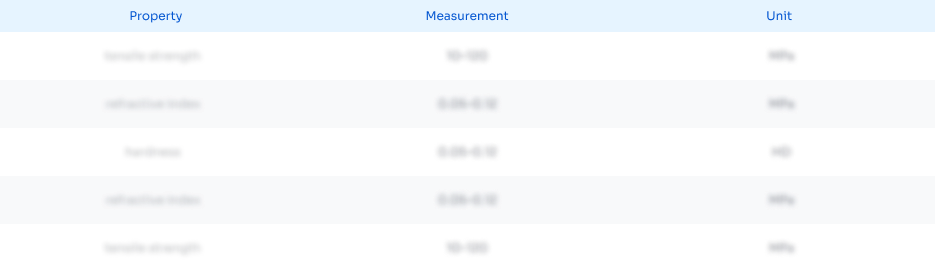
Abstract
Description
Claims
Application Information
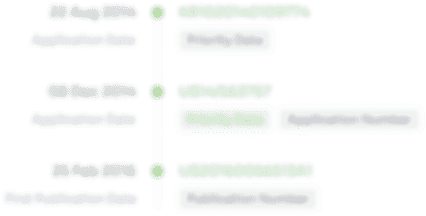
- R&D Engineer
- R&D Manager
- IP Professional
- Industry Leading Data Capabilities
- Powerful AI technology
- Patent DNA Extraction
Browse by: Latest US Patents, China's latest patents, Technical Efficacy Thesaurus, Application Domain, Technology Topic.
© 2024 PatSnap. All rights reserved.Legal|Privacy policy|Modern Slavery Act Transparency Statement|Sitemap