Semi-automatic vegetation remote sensing sample selection method based on convolutional neural network
A convolutional neural network, semi-automatic technology, applied in biological neural network models, neural architectures, instruments, etc., can solve the problems of scarcity of samples in mountain vegetation classification, mountain vegetation remote sensing classification samples, and human training samples. Scarcity of samples and the effect of increasing the degree of automation
- Summary
- Abstract
- Description
- Claims
- Application Information
AI Technical Summary
Problems solved by technology
Method used
Image
Examples
Embodiment Construction
[0040] In order to make the features and advantages of this patent more obvious and easy to understand, the following specific examples are given together with the accompanying drawings and described in detail as follows:
[0041] like figure 2 As shown, the semi-automatic vegetation remote sensing sample selection method based on deep convolutional neural network provided by this embodiment includes the following steps:
[0042] Step S1: Construct a multi-source and multi-temporal remote sensing database using high-resolution remote sensing images and DEM data in spring and autumn;
[0043]Step S2: According to the classification system of the Chinese vegetation map (1:1 million), the vegetation in the study area is divided into m vegetation groups;
[0044] Step S3: According to the number m of vegetation groups in the study area, construct a deep residual network ResNet 18, and change the number of network output categories to m;
[0045] Step S4: Using the public vegeta...
PUM
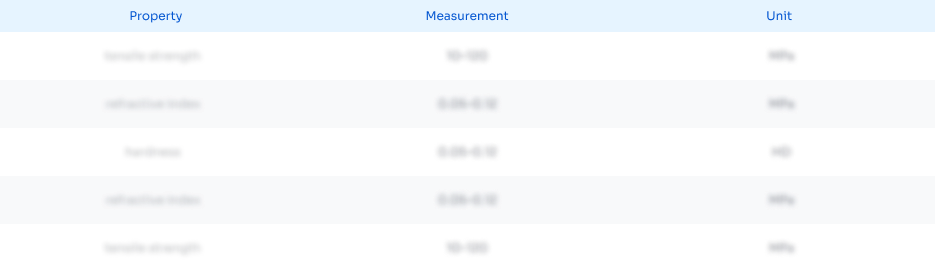
Abstract
Description
Claims
Application Information
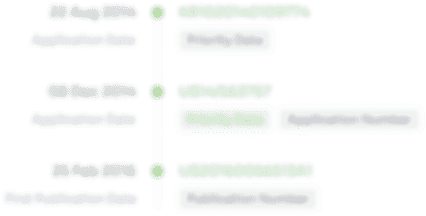
- R&D Engineer
- R&D Manager
- IP Professional
- Industry Leading Data Capabilities
- Powerful AI technology
- Patent DNA Extraction
Browse by: Latest US Patents, China's latest patents, Technical Efficacy Thesaurus, Application Domain, Technology Topic.
© 2024 PatSnap. All rights reserved.Legal|Privacy policy|Modern Slavery Act Transparency Statement|Sitemap