Remote sensing image scene multi-label classification method and device and multi-label retrieval method and device based on graph network
A technology of remote sensing images and classification methods, which is applied in still image data retrieval, still image data clustering/classification, neural learning methods, etc. information and other issues, to achieve the effect of good image features and accurate interpretation
- Summary
- Abstract
- Description
- Claims
- Application Information
AI Technical Summary
Problems solved by technology
Method used
Image
Examples
Embodiment 1
[0058] Embodiment 1: a remote sensing image scene multi-label classification method based on graph network, including:
[0059] Convert the image into a graph structure;
[0060] Using the pre-built and trained multi-scale graph convolution network, based on the graph structure, the multi-label classification is carried out on the ground objects in the image scene, and the multi-label classification results are obtained.
[0061] Building and training a multi-scale graph convolutional network includes:
[0062] Step 1. Construct a scene image library for multi-label classification and retrieval. During specific implementation, the scene image library may be constructed in advance. In the embodiment, firstly, large-scale remote sensing images are segmented with a fixed size to obtain a scene image library, and divided into training sets and the test set Two sub-image libraries, where the training set For network training, test set It is used for multi-label classifica...
Embodiment 2
[0081] Embodiment 2: On the basis of Embodiment 1, this implementation provides a remote sensing image scene multi-label retrieval method based on a graph network (such as figure 1 shown), also includes:
[0082] Using the graph network-based remote sensing image scene multi-label classification method provided in the above embodiments to perform multi-label classification on the query image and the features in each image scene in the image database, and obtain the multi-label classification result;
[0083] Based on the graph structure converted from the query image and the result of multi-label classification, the image library is searched for the first time, and an image set containing at least one of the same features as the query image is obtained;
[0084] Using the pre-built and trained graph similarity network to search the image collection for the second time to obtain the similarity between the query image and other images in the image library, the graph similarity ...
Embodiment 3
[0100] Implementation 3: Corresponding to the graph network-based remote sensing image scene multi-label classification method provided in the above embodiment, this embodiment provides a graph network-based remote sensing image scene multi-label classification device, including a graph structure representation module and a multi-scale graph Convolutional network module;
[0101] The graph structure representation module is used to convert an image into a graph structure;
[0102] The multi-scale graph convolutional network module is used to construct and train the multi-scale graph convolutional network module; the pre-built and trained multi-scale graph convolutional network is used to perform multi-label classification on the ground objects in the image scene based on the graph structure , to obtain the multi-label classification result;
[0103] The multi-scale graph convolutional network module includes respectively established for different scales of the image N +1-lay...
PUM
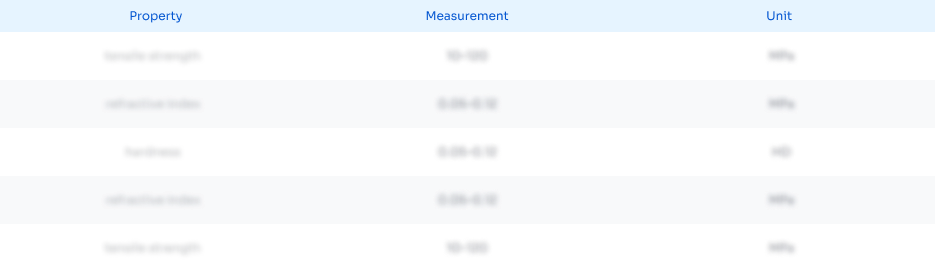
Abstract
Description
Claims
Application Information
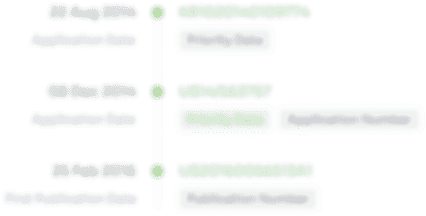
- R&D Engineer
- R&D Manager
- IP Professional
- Industry Leading Data Capabilities
- Powerful AI technology
- Patent DNA Extraction
Browse by: Latest US Patents, China's latest patents, Technical Efficacy Thesaurus, Application Domain, Technology Topic, Popular Technical Reports.
© 2024 PatSnap. All rights reserved.Legal|Privacy policy|Modern Slavery Act Transparency Statement|Sitemap|About US| Contact US: help@patsnap.com