Remote sensing image small target detection method based on improved YOLOv3
A small target detection and remote sensing image technology, applied in the field of deep learning and target detection, can solve problems such as the inability to fully reflect the positional relationship between the two frames, the direction in which the overlapping prediction frames need to move closer, unfavorable search and identification, and many interference factors. To achieve the effect of improving the receptive field, performance improvement, and training speed
- Summary
- Abstract
- Description
- Claims
- Application Information
AI Technical Summary
Problems solved by technology
Method used
Image
Examples
Embodiment
[0072] A small target detection method for remote sensing images based on improved YOLOv3, which specifically includes the following steps:
[0073] Step 1: Obtain training remote sensing images to form a data set, convert the annotation information of the data set into VOC format, and randomly divide it into a training verification set and a test set at a ratio of 9:1. Each set does not interfere with each other, and there are no identical pictures. Prevent data from being polluted. Cross-validation is used during training, that is, the training and verification set is randomly divided into a training set and a verification set at a ratio of 8:1. The training set is used for model training and weight update, and the verification set is used to verify the model obtained at the end of each round of training. Evaluate.
[0074] Step 2: Improve the Neck. With the deepening of the Darknet-53 network, the extracted target features will change from more to less, from concrete to a...
PUM
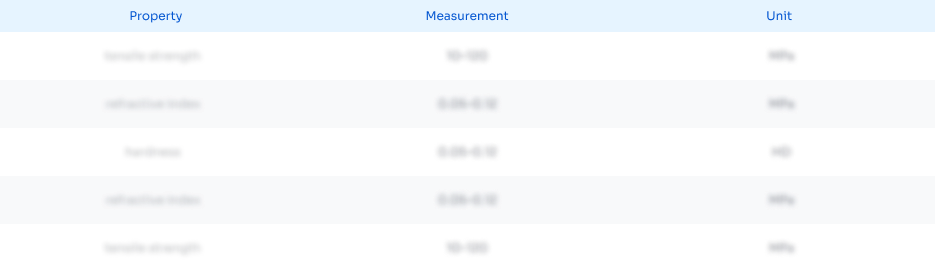
Abstract
Description
Claims
Application Information
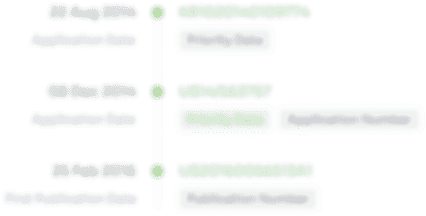
- R&D Engineer
- R&D Manager
- IP Professional
- Industry Leading Data Capabilities
- Powerful AI technology
- Patent DNA Extraction
Browse by: Latest US Patents, China's latest patents, Technical Efficacy Thesaurus, Application Domain, Technology Topic, Popular Technical Reports.
© 2024 PatSnap. All rights reserved.Legal|Privacy policy|Modern Slavery Act Transparency Statement|Sitemap|About US| Contact US: help@patsnap.com