Financial scene-oriented end-to-end natural language processing training framework and method
A natural language processing and financial technology, applied in natural language data processing, finance, data processing applications, etc., can solve the problems of excessive model pressure testing time, time-consuming, huge tasks, etc., to reduce configuration requirements and improve semantic understanding effect of ability
- Summary
- Abstract
- Description
- Claims
- Application Information
AI Technical Summary
Problems solved by technology
Method used
Image
Examples
Embodiment Construction
[0049] The following describes several preferred embodiments of the present invention with reference to the accompanying drawings, so as to make the technical content clearer and easier to understand. The present invention can be embodied in many different forms of embodiments, and the protection scope of the present invention is not limited to the embodiments mentioned herein.
[0050] Such as figure 1 As shown, it is a system block diagram of a preferred embodiment of the present invention, including Google's native BERT module, FinBERT pre-training module, new data mining module from external related data based on similar self-training ideas, and pre-training on downstream task corpus module, using a semi-supervised learning framework to make full use of the unlabeled corpus module, knowledge distillation module and online deployment module.
[0051] Google's native BERT module is the starting point of the entire training framework, including Google's native BERT (Chinese)...
PUM
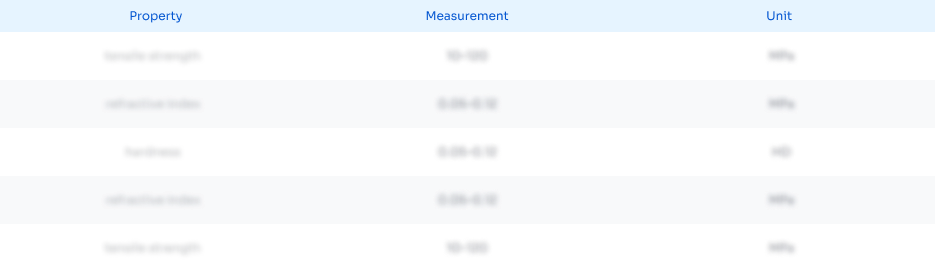
Abstract
Description
Claims
Application Information
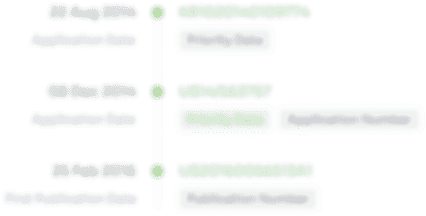
- Generate Ideas
- Intellectual Property
- Life Sciences
- Materials
- Tech Scout
- Unparalleled Data Quality
- Higher Quality Content
- 60% Fewer Hallucinations
Browse by: Latest US Patents, China's latest patents, Technical Efficacy Thesaurus, Application Domain, Technology Topic, Popular Technical Reports.
© 2025 PatSnap. All rights reserved.Legal|Privacy policy|Modern Slavery Act Transparency Statement|Sitemap|About US| Contact US: help@patsnap.com