Lithium ion battery residual life prediction method based on time convolutional neural network
A convolutional neural network, lithium-ion battery technology, applied in neural learning methods, biological neural network models, neural architectures, etc., can solve problems such as affecting computing efficiency, inability to parallel computing, and accelerating training.
- Summary
- Abstract
- Description
- Claims
- Application Information
AI Technical Summary
Problems solved by technology
Method used
Image
Examples
Embodiment Construction
[0045] The method for predicting the remaining life of lithium-ion batteries based on TCN, the specific implementation plan is as follows:
[0046]Step 1: The battery is subjected to repeated charge and discharge experiments at a room temperature of 24°C to collect the influence of battery aging on internal parameters. During the charge and discharge process, charge with a constant current mode of 1.5A until the battery voltage reaches 4.2V, and then continue to charge with a constant voltage mode until the charge current drops to 20mA. Discharging is carried out at a constant current of 2A until it drops to 2.7V, and the charging and discharging process is repeated until the rated capacity drops from 2Ahr to 1.4Ahr. Collect the operating state parameter values of lithium-ion batteries under different working conditions during the whole life cycle operation, including battery terminal voltage, battery output current, battery temperature, load measurement current, load measur...
PUM
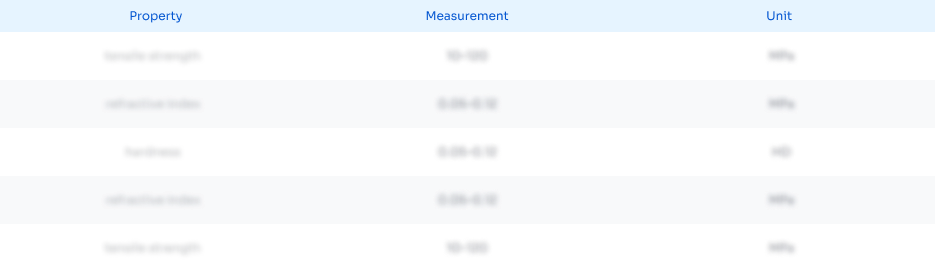
Abstract
Description
Claims
Application Information
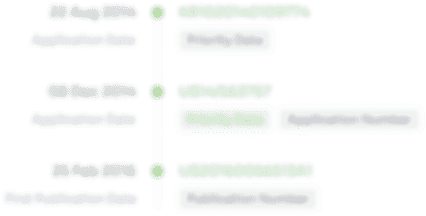
- R&D Engineer
- R&D Manager
- IP Professional
- Industry Leading Data Capabilities
- Powerful AI technology
- Patent DNA Extraction
Browse by: Latest US Patents, China's latest patents, Technical Efficacy Thesaurus, Application Domain, Technology Topic, Popular Technical Reports.
© 2024 PatSnap. All rights reserved.Legal|Privacy policy|Modern Slavery Act Transparency Statement|Sitemap|About US| Contact US: help@patsnap.com