WGAN-GP privacy protection system and method based on improved PATE
A privacy protection system and privacy protection technology, applied in the field of models with comprehensive performance improvement, can solve problems such as unpredictable model impact, model failure to converge, and privacy budget exhaustion, reducing query costs, improving accuracy, and improving consensus. degree of effect
- Summary
- Abstract
- Description
- Claims
- Application Information
AI Technical Summary
Problems solved by technology
Method used
Image
Examples
specific Embodiment approach
[0083] 本发明最终的实现形式是一个基于差分隐私的生成式对抗网络数据隐私保护模型。 利用该模型能对包含敏感信息的训练数据进行差分隐私保护,通过WGAN-GP框架的生成器生成极为逼真的生成样本,为机器学习模型提供不包含真实隐私的训练数据集。 The specific embodiment of the present invention is as follows:
[0084] 通过k折交叉验证法(本发明中k取10)对教师分类模型进行优选。选出具有较好分类效果的n个(m*t=n,t为类别数,m为每个类别块的模型数)分类模型后,将训练数据集划分为n个等大小且不重叠的子数据集,依次分别独立训练每一个优化的教师分类器,组成j个分类块(教研室)。生成器首先从高斯分布中随机采样噪声合成生成样本,每一个分类块下的优化的教师分类器对生成样本做分类预测,将教研室内的优化的教师分类器输出的投票结果聚合,一个分类块输出一个投票结果,通过有条件的差分隐私聚合器对结果进行噪声添加和共识度检查。当通过检查时,对j个分类块的结果噪声聚合后输出一个最终的分类标签,学生判别器通过学习优化的教师分类器集群输出的标签和生成器生成的样本,进行训练,并反向传播优化生成器。当共识度检查未通过时,则放弃输出优化的教师分类器集群对该生成样本的分类结果。如此循环训练,通过对抗博弈的方式优化生成器和学生判别器。当隐私预算用尽或模型训练完成时,可产生不包含任何真实敏感信息的生成样本集。
PUM
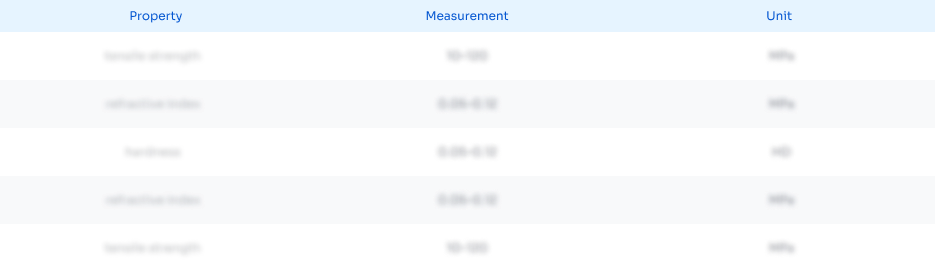
Abstract
Description
Claims
Application Information
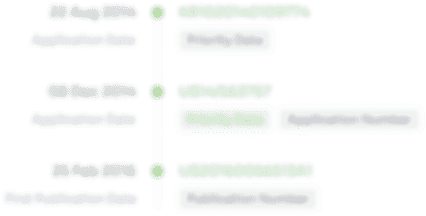
- R&D Engineer
- R&D Manager
- IP Professional
- Industry Leading Data Capabilities
- Powerful AI technology
- Patent DNA Extraction
Browse by: Latest US Patents, China's latest patents, Technical Efficacy Thesaurus, Application Domain, Technology Topic.
© 2024 PatSnap. All rights reserved.Legal|Privacy policy|Modern Slavery Act Transparency Statement|Sitemap