Containerization test method and system for reinforcement learning model
A technology that reinforces learning and testing methods, applied in software testing/debugging, instrumentation, error detection/correction, etc., can solve problems such as dependencies, poor isolation of the testing process, and high testing environment requirements, to ensure fairness, achieve visualization, The effect of ensuring security and privacy
- Summary
- Abstract
- Description
- Claims
- Application Information
AI Technical Summary
Problems solved by technology
Method used
Image
Examples
Embodiment Construction
[0083] The present invention will be described in further detail below in conjunction with the accompanying drawings, and the examples given are only used to explain the present invention, and are not intended to limit the scope of the present invention.
[0084] In this embodiment, the unmanned vehicle decision-making control algorithm in automatic driving is used as a test agent (reinforcement learning model). The testing party refers to the subject that tests and evaluates the decision-making control algorithm of the unmanned vehicle, and provides the Docker image file including the test environment and the test agent template (decision-making control algorithm of the unmanned vehicle) for the test of the decision-making control algorithm of the unmanned vehicle. Unmanned vehicle decision-making control algorithm and test results; the test platform is a management platform for unmanned vehicle decision-making control algorithm testing. The division of the party is used to r...
PUM
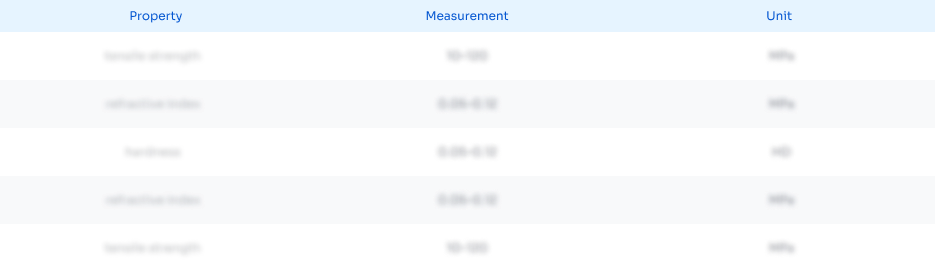
Abstract
Description
Claims
Application Information
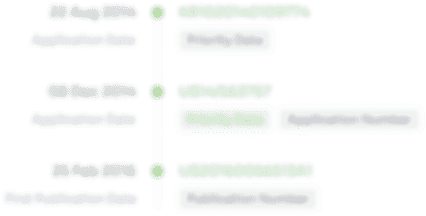
- R&D Engineer
- R&D Manager
- IP Professional
- Industry Leading Data Capabilities
- Powerful AI technology
- Patent DNA Extraction
Browse by: Latest US Patents, China's latest patents, Technical Efficacy Thesaurus, Application Domain, Technology Topic.
© 2024 PatSnap. All rights reserved.Legal|Privacy policy|Modern Slavery Act Transparency Statement|Sitemap