Heavy landing analysis method and device based on a multi-branch time convolutional network
A convolutional network and re-landing technology, applied in the field of re-landing, can solve the problems of high professional barriers, low prediction/classification accuracy, incomplete compiled data, etc., achieve good theoretical and application value, and improve prediction accuracy Effect
- Summary
- Abstract
- Description
- Claims
- Application Information
AI Technical Summary
Problems solved by technology
Method used
Image
Examples
Embodiment Construction
[0060] Embodiments of the present application are described in detail below, examples of which are shown in the accompanying drawings, wherein the same or similar reference numerals denote the same or similar elements or elements having the same or similar functions throughout. The embodiments described below by referring to the figures are exemplary and are intended to explain the present application, and should not be construed as limiting the present application.
[0061] The analysis method and device for heavy landing based on the multi-branch temporal convolutional network of the embodiment of the present application are described below with reference to the accompanying drawings.
[0062] figure 1 It is a flow chart of a heavy landing analysis method based on a multi-branch temporal convolutional network provided in Embodiment 1 of the present application.
[0063] Such as figure 1 As shown, the analysis method of heavy landing based on multi-branch temporal convolu...
PUM
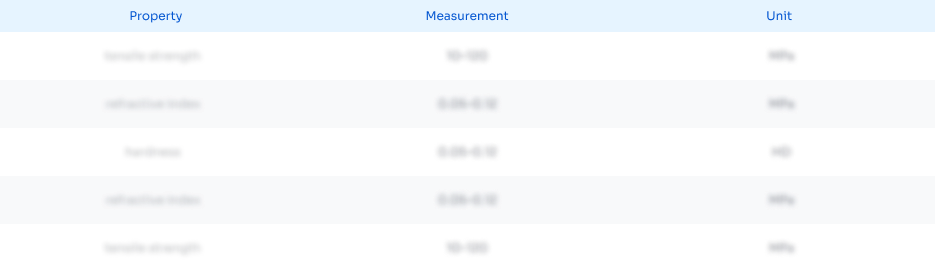
Abstract
Description
Claims
Application Information
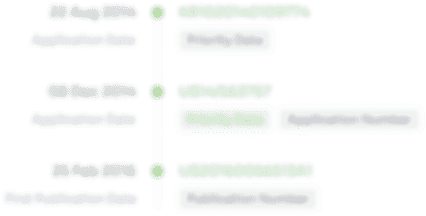
- R&D Engineer
- R&D Manager
- IP Professional
- Industry Leading Data Capabilities
- Powerful AI technology
- Patent DNA Extraction
Browse by: Latest US Patents, China's latest patents, Technical Efficacy Thesaurus, Application Domain, Technology Topic, Popular Technical Reports.
© 2024 PatSnap. All rights reserved.Legal|Privacy policy|Modern Slavery Act Transparency Statement|Sitemap|About US| Contact US: help@patsnap.com