Land cover classification method based on deep fusion of multi-modal remote sensing data
A technology of remote sensing data and land surface coverage, applied in the field of remote sensing, can solve problems such as more exploration in the field of depth feature fusion, and achieve the effect of broad application prospects
- Summary
- Abstract
- Description
- Claims
- Application Information
AI Technical Summary
Problems solved by technology
Method used
Image
Examples
Embodiment Construction
[0052] The present invention will be further described below in combination with specific embodiments. It should be understood that these examples are only used to illustrate the present invention and are not intended to limit the scope of the present invention. In addition, it should be understood that after reading the content taught by the present invention, those skilled in the art may make various changes or modifications to the present invention, and these equivalent forms also fall within the scope defined by the appended claims of the present application.
[0053] A land cover classification method based on deep fusion of multimodal remote sensing data, the steps are as follows:
[0054] (1) Build a model;
[0055] The model is a remote sensing image semantic segmentation network based on multimodal information fusion. The model structure is as follows: figure 1 As shown, it includes an encoder for extracting feature features, a deep feature fusion module, a spatial ...
PUM
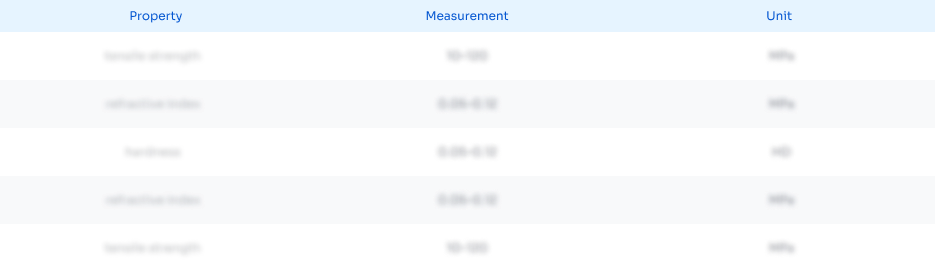
Abstract
Description
Claims
Application Information
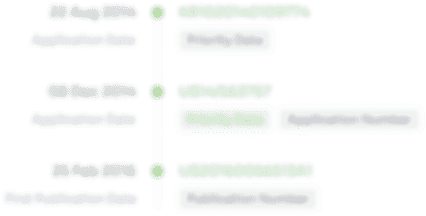
- R&D Engineer
- R&D Manager
- IP Professional
- Industry Leading Data Capabilities
- Powerful AI technology
- Patent DNA Extraction
Browse by: Latest US Patents, China's latest patents, Technical Efficacy Thesaurus, Application Domain, Technology Topic, Popular Technical Reports.
© 2024 PatSnap. All rights reserved.Legal|Privacy policy|Modern Slavery Act Transparency Statement|Sitemap|About US| Contact US: help@patsnap.com