Data distributed incremental learning method, system and device and storage medium
A technology of incremental learning and data distribution, applied in machine learning, computing models, computing, etc., can solve problems such as inability to handle complex scenarios, and achieve the effect of improving learning ability and strong practicability
- Summary
- Abstract
- Description
- Claims
- Application Information
AI Technical Summary
Problems solved by technology
Method used
Image
Examples
Embodiment 1
[0076] refer to figure 1 and figure 2 , the data distributed incremental learning method of the present invention comprises the following steps:
[0077] 1) Determine the number of data distribution nodes and the number of incremental learning stages;
[0078] 2) Establish a training data set;
[0079] 3) Determine the category of each incremental learning stage, divide the training data set into T independent data sets, one of which corresponds to a data set in the incremental learning stage, and then in the current incremental learning stage, according to the corresponding The data collection of each data distribution node is established;
[0080] 4) Input the global shared model parameters of the previous incremental learning stage and the data collection of each data distribution node in the current incremental learning stage to each data distribution node, and then perform incremental learning training under the constraints of the incremental learning loss function, ...
Embodiment 2
[0130] Embodiment 2 A distributed incremental learning system for data, including:
[0131] A determination module is used to determine the number of data distribution nodes and the number of incremental learning stages;
[0132] Build a module for building a training data set;
[0133] The division module is used to determine the category of each incremental learning stage, divide the training data set into T independent data sets, and one incremental learning stage corresponds to a data set, and then in the current incremental learning stage, according to the incremental The data set corresponding to the learning phase establishes the data set of each data distribution node;
[0134] The model construction module is used to input the global shared model parameters of the last incremental learning stage and the data collection of each data distribution node in the current incremental learning stage to each data distribution node, and then increase the value under the constra...
Embodiment 3
[0141] A computer device, comprising a memory, a processor, and a computer program stored in the memory and operable on the processor, when the processor executes the computer program, the distributed incremental learning of the data is realized method steps.
PUM
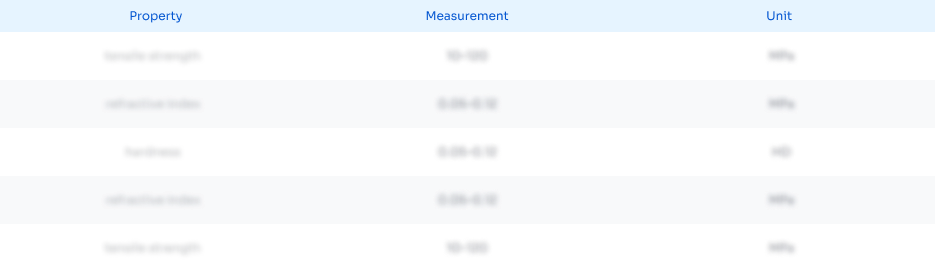
Abstract
Description
Claims
Application Information
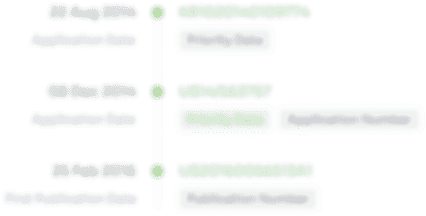
- R&D Engineer
- R&D Manager
- IP Professional
- Industry Leading Data Capabilities
- Powerful AI technology
- Patent DNA Extraction
Browse by: Latest US Patents, China's latest patents, Technical Efficacy Thesaurus, Application Domain, Technology Topic.
© 2024 PatSnap. All rights reserved.Legal|Privacy policy|Modern Slavery Act Transparency Statement|Sitemap